In today’s crowded marketplace, offering a personal shopping experience isn’t just a nice-to-have – it’s a must. An AI product recommendation engine powered by generative AI solutions helps brands deliver tailored suggestions that truly connect with customers by aligning with each person’s unique preferences, behaviors, and browsing patterns. Rather than generic options, these AI product recommendation engines give real-time, personalized recommendations that deepen engagement and foster lasting loyalty.
Generative AI can take recommendations to the next level. With Natural Language Processing, the AI product recommendation engine understands specific customer needs, even from casual queries like “lightweight hiking shoes for summer,” and delivers spot-on results. Learning from real-time user interactions, it continually adapts each session, creating a seamless, scalable experience across both web and mobile.
Why Miquido?
With Miquido’s AI Kickstarter framework, you can implement a custom, AI product recommendation engine designed specifically for e-commerce – quickly and affordably. Leveraging advanced models like GPT-4, Mistral, and Claude, plus Retrieval-Augmented Generation, this solution provides highly personalized product recommendations that adjust dynamically to each shopper’s needs.
Our custom-made engines integrate easily with major eCommerce platforms like Shopify, Magento, and WooCommerce, allowing you to increase engagement and drive sales where it matters. Plus, they’re fully compatible with cloud platforms like AWS, Azure, and GCP, ensuring secure, scalable data handling to support your growth.
Who it’s for:
- eCommerce platforms looking to boost user engagement and sales with personalized product suggestions.
- Retailers and marketplaces aiming to optimize product discovery for users.
- Media and content streaming services seeking to enhance user experience through tailored recommendations.
- Travel and hospitality companies interested in providing personalized travel packages or experiences.
- B2B platforms that require customized recommendations for products and services.
Challenges of traditional product recommendation engines
Traditional product recommendation systems often rely on static algorithms or basic filtering, which can lead to:
- Limited personalization: Many traditional systems are unable to factor in complex, real-time insights from user behavior, leading to less relevant suggestions.
- Low engagement and conversion rates: Generic or repetitive product recommendations often fail to capture user interest, resulting in reduced engagement and lower conversion.
- Manual configuration and maintenance: Standard systems often require significant manual input to categorize and tag products, increasing operational costs.
- Inability to adapt to changing user preferences: Traditional systems struggle to keep up with evolving consumer behavior, especially in fast-paced retail or media environments.
Benefits of the AI product recommendation
The AI product recommendation engine based on generative AI and machine learning has all it needs to overcome these limitations, delivering an engaging and personalized shopping experience. It elevates the entire shopping experience, from discovery to purchase, driving engagement, conversions, and retention with every interaction.
Key benefits include:
- High-level personalization: By analyzing real-time user data, preferences, and purchase history, the Artificial Intelligence product recommendation engine creates tailored recommendations that resonate with each individual customer.
- Improved conversion: Personalized suggestions keep users engaged, often leading to higher conversion rates as customers quickly find what they need.
- Real-time adaptability: The AI product recommendation engine dynamically adjusts recommendations as user preferences shift, maintaining continuous relevance.
- Enhanced product discovery: AI-driven insights help users discover new, relevant products they might otherwise miss, boosting customer loyalty and average order value.
- Cross-platform experience: The engine can integrate effortlessly across web, mobile, and app platforms, delivering a consistent and cohesive shopping experience across all channels.
- Scalable for growth: The AI recommendation engine can scale with your business, ensuring reliable performance for an expanding customer base.
- Efficient inventory management: By analyzing demand patterns, the AI product recommendation engine helps anticipate product needs, supporting better stock management and minimizing overstock or stockouts – enhancing operational efficiency and customer satisfaction.
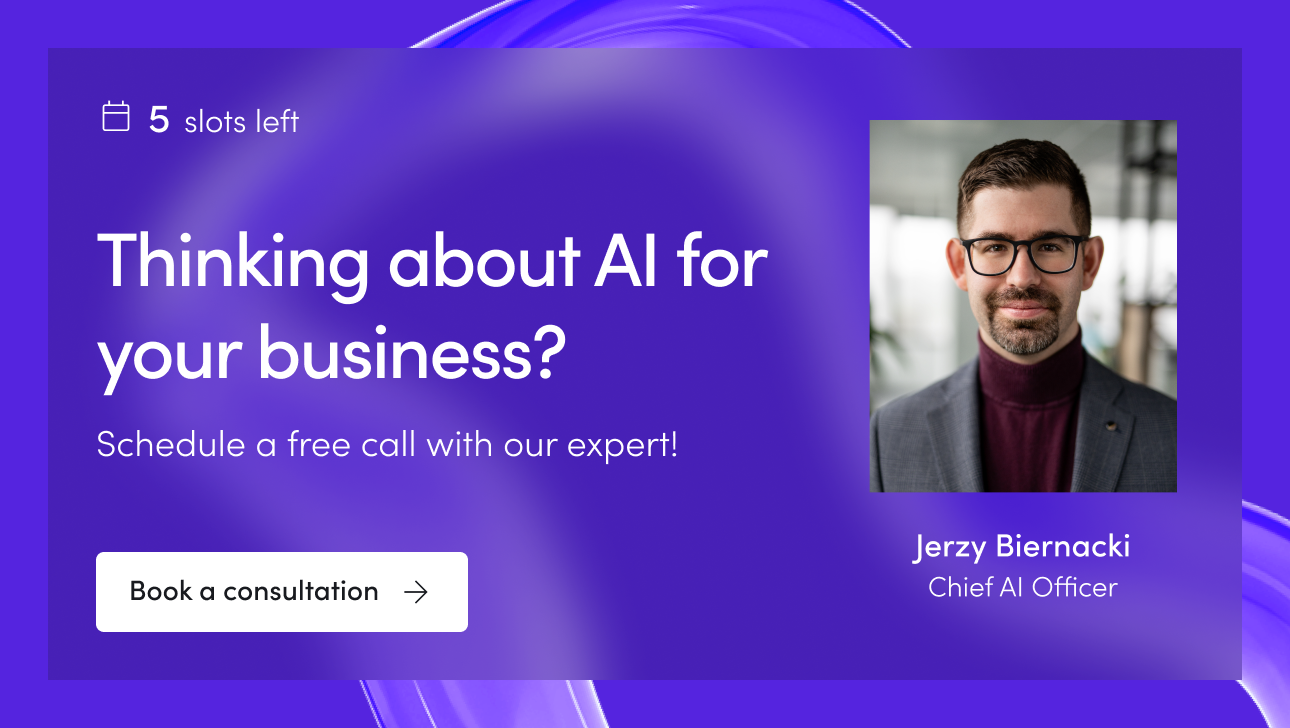
AI product recommendation engine workflow
- Data collection & user profiling
- Data gathering: The AI product recommendation engine can collect nuanced data through users’ natural conversations with the AI assistant. This conversational approach captures details like preferred styles, budget, and specific needs that typical browsing doesn’t reveal.
- Profiling: Data from interactions, browsing history, and past purchases creates a detailed, continuously updated user profile.
- Conversational interaction & contextual understanding
- User engagement: Thanks to NLP, users can describe what they’re looking for conversationally (e.g., “gift for a hiking enthusiast”). This approach feels intuitive and guides relevant product recommendations.
- Contextual insights in real time: The AI product recommendation engine can retrieve and generate recommendations based on conversation context, refining suggestions on the fly to ensure they’re aligned with user needs.
- Personalized recommendation
- Real-time customization: Feedback and interactions shape recommendations in real time, adapting to individual preferences and seasonal trends to keep suggestions fresh.
- Omni-channel delivery: Recommendations can reach users consistently across web, mobile, email, and chat, enabling a seamless shopping experience on any platform.
- Feedback loop
- Learning from data: Each interaction strengthens the engine’s relevance, based on user response to recommendations. Regular updates can incorporate new data and trends, keeping recommendations aligned with evolving preferences and market shifts for long-term engagement and loyalty.
AI product recommendation engine features
- Contextual understanding: Thanks to NLP, the AI product recommendation engine can interpret complex customer data, such as specific preferences or combination searches (e.g., “eco-friendly running shoes”).
- Real-time updates: Recommendations update dynamically with every interaction, ensuring suggestions are timely and relevant to current customer behavior.
- Multi-channel personalization: An advanced product recommendation engine delivers relevant recommendations through web, email, mobile, and chat interfaces, providing users with a seamless, personalized experience across all platforms.
- Advanced categorization: The AI product recommendation engine can also leverage machine learning to automatically categorize products, eliminating the need for manual tagging and allowing for more accurate, personalized filtering.
- AI-powered A/B testing and optimization: The engine can continually optimize recommendation strategies through AI-driven testing, identifying configurations that maximize engagement and conversions.
Case studies
An eCommerce company collaborated with Miquido to create an AI recommendation chatbot that delivers personalized product suggestions. This intelligent system analyzes user input, goals, and customer preferences to provide accurate, context-aware recommendations that enhance the shopping experience.
To support this, Miquido developed a comprehensive knowledge base, aggregating product details and domain-specific insights. The chatbot was integrated seamlessly into the company’s eCommerce platform, driving user engagement, increasing conversion rates, and boosting customer satisfaction through tailored recommendations – all while addressing data privacy and regulatory compliance.
Cost structure
With the AI Kickstarter framework, building an advanced AI-powered product recommendation engine starts at $15,000, which covers:
- Initial setup: Includes data ingestion, AI Kickstarter setup, and customization to align the recommendation engine with the unique needs and data sources of your business.
- Maintenance: Ensures the engine stays accurate and relevant, continuously learning from new user interactions, product changes, and market trends.
- Optional customization: Available for businesses that require specialized integrations, compliance features, or unique recommendation criteria to meet industry standards.
This flexible cost structure allows organizations to implement a robust, scalable AI product recommendation engine that adapts to their business needs.
ROI
Businesses typically see a return on investment within 3 months of implementation, driven by increased customer engagement, higher conversion rates, and improved customer retention. The AI product recommendation engine’s ability to deliver highly personalized, real-time suggestions enhances user experience and drives sales, making it a powerful tool for achieving measurable business growth.