In the ever-evolving landscape of financial technology (FinTech), data has emerged as the new currency. It drives innovation and reshapes the industry. With the rapid proliferation of digital transactions, online banking, and mobile payments, a staggering amount of data is generated every second.
However, not just the data volume matters but how the financial firms and institutions harness its potential to gain valuable insights and drive informed decision-making.
The scale of information circulating through the physical world and the global economy is immense and diverse. It originates from various channels like sensors, satellite images, web activity, digital applications, videos, and credit card transactions.
However, the unprocessed data has limited significance until it is combined with other data and converted into information to enhance decision-making. The data gains relevance and reveals valuable insights by contextualising, for example, sales figures within historical data or market trends. Suddenly, these figures can indicate growth or decline compared to benchmarks or as a result of specific strategies.
As the volume of data generated from financial transactions continues to surge, it becomes imperative for FinTech companies to invest in data analytics and the integration of predictive analytics supported by AI. To harness the full potential of these vast datasets and enable advanced data analysis, they often seek the expertise of AI development services. Especially those which employ algorithms and machine learning techniques to extract and identify patterns and drive informed decision-making processes.
Introduction to AI in FinTech
According to the Mordor Intelligence report, the AI in Fintech Market size is estimated at $42.83 billion in 2023. It is expected to reach $49.43 billion by 2028. Furthermore, it is anticipated to grow at a CAGR of 2.91% during the forecast period (2023-2028).
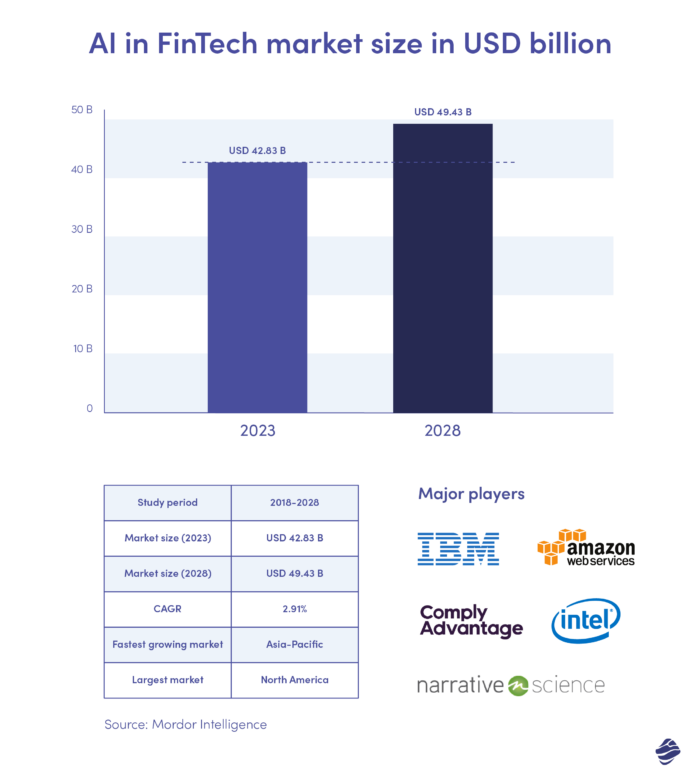
That’s proof that with predictive analytics, banking and financial institutions can gain valuable insights into future trends, customer behaviour, and market dynamics to make proactive, informed, strategic business decisions.
What’s more, the recent Mckinsey’s report “Autonomy of AI: Staying on the forefront of AI in banking” highlights the importance of technology, particularly AI, blockchain, cloud computing, and data and predictive analytics tools, in achieving efficiency and driving business innovation in the financial industry. These technologies allow banking companies to serve a vast customer base, including millions of individual customers and business clients, with remarkable efficiency on their digital platform. The low per-account IT operational and maintenance cost further adds to their advantage in serving the mass population.
Leveraging cloud computing and data analytics enables the financial services industry to develop superior risk models. It leads to the swift assessment of financial risks and approval of credit products for customers, a previously unimaginable process.
Moreover, financial companies can create a commercially sustainable and profitable business model by successfully processing credit requests and maintaining a balance between costs and returns. The highlighted examples showcased how embracing technology-driven solutions can transform traditional finance processes and banking practices, making financial services more accessible and efficient for a broader audience.
Advanced Data Analytics for improved decision-making
Implementing AI-driven insights enables FinTech businesses to establish a comprehensive data strategy encompassing descriptive, predictive, and prescriptive analytics. Machine learning and AI implementation widen the capabilities and ensure more data confidence for each type of these analytical approaches.
Nowadays, financial organisations follow suit by automating data processing through the utilisation of analytics automation tools and predictive modelling. Depending on the business perspective, the data strategy for the finance team can consist of the following:
- Descriptive analytics, which involves transforming raw data, such as sales figures, counts, and customer statistics, into actionable information that can guide decision-making. FinTech businesses can gain insights into past performance and trends by analysing historical data.
- Predictive analytics leverages advanced technologies like AI, machine learning, and Big Data to forecast future events and outcomes. By identifying patterns and correlations within data, predictive analytics enables businesses to make informed predictions about future trends, customer behaviour, and market dynamics.
- Prescriptive analytics furthers the analysis by providing intelligent recommendations and suggesting optimal courses of action to achieve desired outcomes. By considering various data inputs and using algorithms, prescriptive analytics assists businesses in addressing challenges and making data-driven decisions.
Prescriptive and predictive analytics offers extensive insights into the FinTech business’s issues. They provide guidance on the most effective strategies for addressing them. These analytics methodologies enable businesses to optimise performance, drive efficiency, and capitalise on opportunities in a rapidly evolving marketplace.
Another type of analytics with a significant importance in the banking and financial sector is real-time. Real-time analytics refer to the process of analysing data and statistical models and generating insights in real-time or near real-time. It involves continuously monitoring and processing data streams as they are generated, enabling immediate decision-making and action for FinTech institutions.
Benefits of AI-Driven Insights in FinTech
Among the so-called “edge technologies” falling under AI-powered ones are natural language processing (NLP), voice-script analysis, virtual agents, computer vision, facial recognition, blockchain, robotics, and behavioural analytics. These advanced capabilities play a pivotal role in enhancing customer experience and loyalty through various aspects such as engagement channels, intelligent advisory, and faster processing. Additionally, they enable personalised offers with precise underwriting. Last but not least, they drive operational efficiency throughout the entire value chain. They encompass customer servicing, monitoring, record management, and beyond.
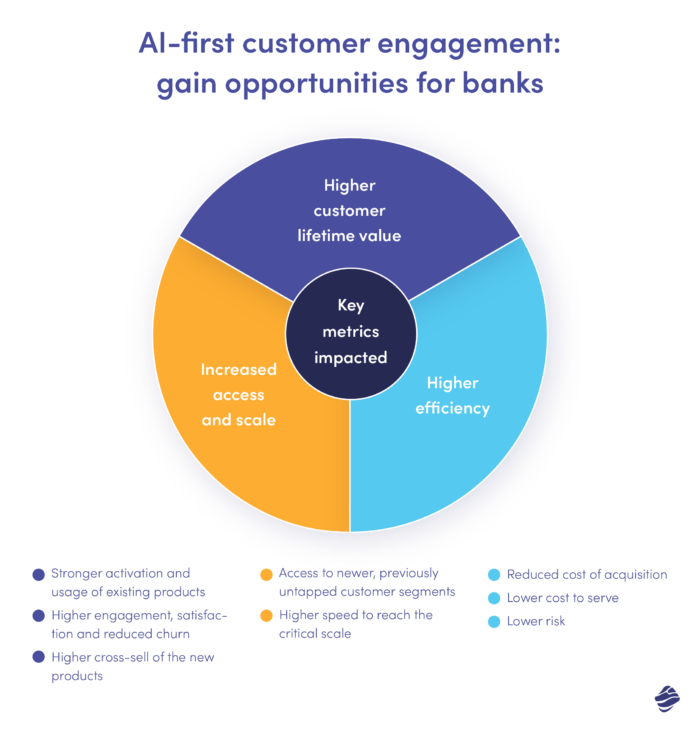
According to a McKinsey report, by prioritising AI/ML use cases, financial institutions can achieve automation for over 20 decision-making processes across various customer journeys. Notably, banks that are increasingly turning to AI and analytics capabilities can enhance key areas:
- customer acquisition,
- credit decisions,
- monitoring and collections,
- strengthening customer relationships,
- and intelligent servicing.
Several benefits of incorporating predictive capabilities into artificial intelligence and machine learning in the finance sector include:
- Enhanced Customer Acquisition: By employing end-to-end automation and advanced analytics, banks can create exceptional customer experiences and deliver highly personalised messages throughout the customer acquisition journey. This approach gives them a competitive advantage, leading to stronger customer acquisition rates.
- Increased Customer Lifetime Value: Continuously and intelligently engaging with customers across a range of products and services strengthens the customer relationship, ultimately increasing their lifetime value to the bank.
- Improved Operating Efficiency: Embracing automation extensively, especially in document processing, review, and decision-making stages during acquisition and servicing, allows banks to lower their operating costs significantly.
- Reduced Credit Risk: Banks can effectively mitigate credit risks by more sophisticated screening of potential customers and early detection of behaviours signalling higher risk of default and fraud, ensuring a more stable and secure financial environment.
Moreover, through the implementation of AA/ML models capable of analysing extensive and varied datasets in almost real-time, banks can efficiently assess new customers for credit services, establish appropriate loan limits and pricing, and gain better visibility into future outcomes of cash inflows and outflows with cash flow forecasting features.
Use cases of Predictive Analytics in financial services
Predictive analytics, a facet of advanced analytics, harnesses historical data, statistical modelling, data mining, and machine learning to anticipate forthcoming outcomes.
Finance leaders widely embrace predictive analytics in finance to discern patterns in data, enabling the identification of potential risks and opportunities. Often aligned with big data and data science, contemporary companies grapple with a deluge of data ranging from log files to images and videos scattered across diverse repositories within the organisation. Therefore, data scientists can deploy deep learning and machine learning algorithms to extract these valuable insights from information reservoirs, effectively discerning patterns and forecasting future events. Prominent statistical techniques like logistic and linear regression models, neural networks, and decision trees form the bedrock of this predictive process, generating preliminary predictive learnings that fuel further predictive insights and financial decisions. Below, we will explore several use cases of how predictive analytics can be used in financial services below.
Credit Scoring and risk assessment in Finance
Banks use a predictive model and analytics to evaluate the creditworthiness of potential borrowers. By analysing historical data, credit scores, financial behaviour, and other relevant factors, predictive models can assess the likelihood of loan default or repayment, enabling banks to make informed lending decisions.
How do banks handle credit risk management?
Banks employ several tactics to manage credit risk effectively. They establish precise lending criteria, which may include mandating a minimum credit score for borrowers. Additionally, they routinely monitor their loan portfolios, evaluating fluctuations in borrowers’ creditworthiness and making necessary modifications as required.
To assess the risk cost, lenders utilise a wide range of data from the borrower, the lender themselves, and external entities like credit agencies. Credit scores and credit risk analysis models are among the methods employed, enabling lenders to estimate the anticipated loss based on factors such as the probability of default, loss-given default, and exposure at default.
Leveraging AI-powered credit risk analysis enables lenders to make more informed decisions about loan applications, detect subtle patterns, and predict potential defaults with greater precision, ultimately reducing risks and enhancing the overall loan portfolio quality.
How optimising credit scoring can substantially boost your revenue?
Miquido’s invited the CEO of NextBank to discuss the practical applications of AI and pioneering advancements in FinTech.
In a free webinar centred around applying AI in the financial and banking business, we focused on practical applications of AI and how to transform your credit scoring into a profitable powerhouse. The webinar presents a mobile banking case study of NextBank.
Fraud Detection using AI and ML
Financial institutions can employ predictive analytics to detect fraudulent activities in real-time. By continuously analysing transactional data and customer behaviour patterns, banks can flag suspicious transactions and prevent fraudulent activities, safeguarding both customer assets and the bank’s reputation.
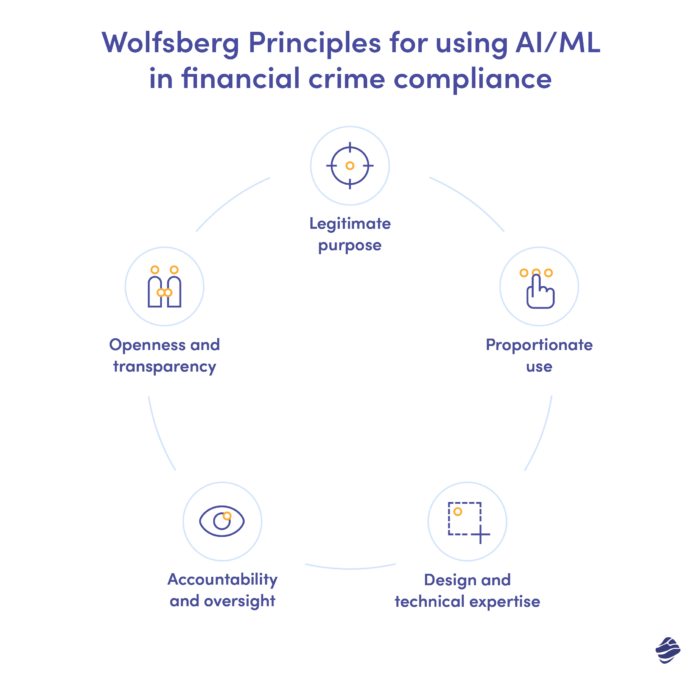
In 2022, the Wolfsberg Group, an association of 13 global banks that aims to develop frameworks and guidance for managing financial crime risks, has outlined five best practices to ensure the responsible use of AI and ML in managing financial crime risk.
These practices include clearly defining the scope of AI tools and implementing governance plans to mitigate the risk of misuse while considering data misappropriation and algorithmic bias. It emphasises the need for proportional use of AI’s power, with regular risk assessments to balance benefits like risk-based alert prioritisation and fraud detection. What’s more, adequate design and technical expertise are essential to understand AI’s functions, define objectives, control for drawbacks like bias, and ensure explainability for investigators.
The framework also emphasises accountability and oversight throughout AI’s lifecycle, even when using vendor-provided AI, with ethical checks and compliance alignment. Striking a balance between regulators’ transparency expectations and confidentiality requirements is crucial, and AI systems should provide clear reasons for risk detection decisions to maintain openness and ensure a robust audit trail.
Protecting banking clients from potential fraud attempts: a case study of NextBank
NextBank, a forward-thinking banking company, recognised the potential of a secure and user-friendly mobile app. Committed to providing its clients with unparalleled security and convenience, NextBank created a mobile app that not only met stringent security requirements but also delivered a seamless banking experience.
NextBank adopted a multi-layered approach that incorporated the latest security protocols and industry best practices. Implementing end-to-end encryption and stringent authentication procedures, they instilled a sense of trust among users, encouraging them to conduct financial transactions with peace of mind.
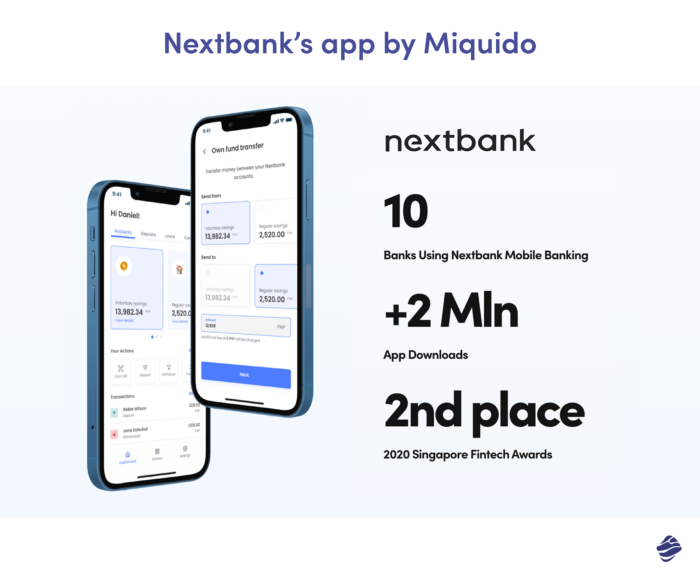
To comply with strict OWASP Application Security Verification Standards, the development team at Nextbank adopted a cost-effective, secure banking app. This ensured the highest data protection and security standards. NextBank’s case study demonstrates their extra efforts to protect clients from potential fraud attempts.
AI-powered customer segmentation and personalisation in finance
Predictive analytics is used to segment customers based on their preferences, behaviours, and needs. By understanding customer segments, banks can offer personalised financial products and services, enhance customer experiences, and drive customer loyalty. However, banking and financial organisations bear the responsibility of devising strategies that fulfil numerous segmentation goals without violating compliance policies and established legal terms.
Financial institutions can take advantage of segmentation and personalisation initiatives across sales and marketing channels by creating a cohesive narrative based on data from multiple campaigns and touchpoints. Secondly, creating personalised blog content targeted towards small customer segments based on product usage and interest. Finally, segmenting customers based on their buying cycle and tailoring marketing efforts to suit their needs at each journey stage. These approaches involve marketing analytics and data-driven content creation to optimise customer experiences and strengthen relationships.
LLMs and AI will impact the user experience in the banking industry in multiple ways.
Forbes The Future Of AI In Banking
Examples include analysing customer data to offer personalised customer service, customised financial advice, and proactive fraud detection. Moreover, AI can help automate routine tasks, increasing efficiency and reducing costs for banks while providing faster customer support. Lastly, AI can aid in creating a conversational banking experience. It is second to none when it comes to simplifying user interactions and making banking services available in multiple languages.
AI-enhanced personalization in SBAB’ app: a Miquido’s case study
The SBAB mobile app, developed by Miquido, is a comprehensive platform for financial savings and loan management.
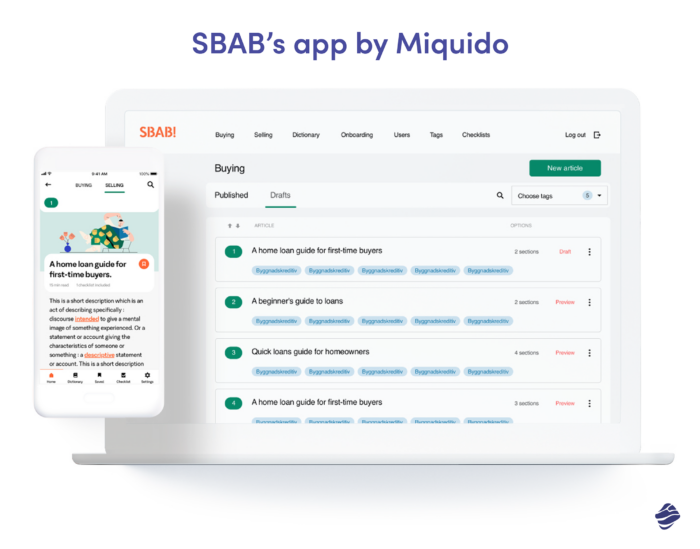
As a prime example of AI-powered personalisation, Miquido integrated intuitive mortgage loan guides for home buyers within the app. The SBAB app offers users convenient access to frequently sought-after information about mortgage loans, streamlining potential home buyer’s decision-making process.
Churn Prediction and Customer Retention
Predictive models help banks identify customers who are likely to churn or close their accounts. The churn prediction algorithm can leverage a variety of factors for predictive analytics in finance. The noteworthy factors include customer demographics and psychographics, transactions, pricing data, economic factors, customer behaviour elements, and customer journeys.
Banks can retain valuable customers and reduce churn rates by intervening proactively and offering tailored incentives or personalised offers.
Market trend analysis and investment opportunities
With the help of predictive analytics, financial organisations can analyse market trends and forecast changes in market conditions. These insights aid in making strategic investment decisions, adjusting portfolios, and capitalising on emerging opportunities.
What’s more, predictive analytics lets financial organisations identify investment opportunities by analysing historical data, market trends, and customer behaviour. By understanding the dynamics of various financial instruments and asset classes, organisations can make data-driven investment choices. They can also capitalise on emerging trends, and optimise their portfolios to achieve better returns.
Cross-Sell and Upsell Opportunities
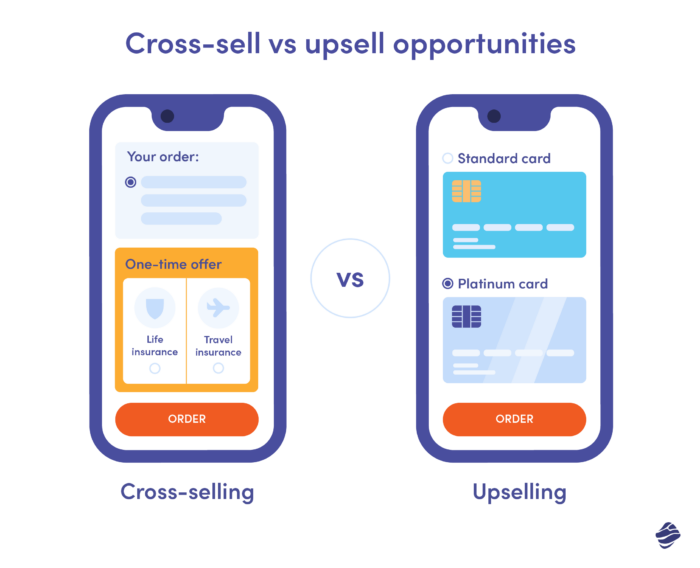
Predictive analysis helps banks identify cross-selling and upselling opportunities for existing customers. By analysing customer data and purchase history, banks can recommend relevant financial products and services. And, as a result, increase revenue and customer engagement.
Portfolio Optimization
Predictive models use risk appetite, historical market data, and client objectives to recommend optimal asset allocations for improved investment portfolios. Portfolio optimisation has key advantages for investors and managers. It aims to maximize returns while effectively managing risk, achieving a desirable risk-return trade-off on the efficient frontier. This satisfies clients by delivering high returns per unit of risk.
Secondly, portfolio optimisation emphasises diversification, reducing unsystematic or non-priced risks and protecting investors from the downsides of underperforming assets. A well-diversified portfolio ensures investors remain in a comfortable zone even in turbulent market conditions. Furthermore, active portfolio management and the analysis of market data enable managers to identify market opportunities ahead of others. It also allows them to capitalise on these opportunities for the benefit of their investors.
Regulatory Compliance
Predictive analytics assists banks in ensuring regulatory compliance. Banks can avoid penalties and meet regulatory requirements by analyzing vast data. They use this data to identify potential compliance risks and take preemptive measures.
The emergence of AI has led to enhanced efficiency, accuracy, and cost-effectiveness in compliance processes within the financial services sector.
Jon Leitner President at Ascent
AI-powered tools can efficiently organise large volumes of data and documents by categorisation, tagging, and indexation. This capability is particularly beneficial for regulatory compliance. It enables financial institutions to swiftly identify and monitor specific transactions, customers, or behavioural patterns. Additionally, AI’s ability to standardise data structure removes subjectivity, ensuring consistency across different departments and facilitating easy comparisons. As a result, the potential for errors or omissions in compliance reports is significantly reduced.
Predictive Models in Financial Forecasting
Predictive models have transformed financial forecasting into a dynamic and data-driven discipline, empowering businesses with insights that were previously inaccessible. These models are indispensable tools for businesses and finance professionals. They help with revenue forecasting, credit risk assessment, and understanding clients in an unpredictable financial landscape.
The role of AI in financial forecasting and decision-making
AI’s ability to process vast volumes of data, uncover intricate patterns, and generate predictions has drawn attention from industry leaders. Experts emphasize AI’s potential to enhance financial forecasting by enabling more precise and real-time insights. Additionally, AI’s role increases in augmenting human decision-making. When combined with human expertise, AI can significantly improve decision outcomes in complex and data-intensive scenarios.
By harnessing AI-powered predictive analytics, financial institutions gain a competitive edge. AI-driven forecasts provide critical insights into market trends, consumer behaviour, supply chains, and risk management. They also allow for agile and well-informed decision-making. This combination of human expertise with AI’s analytical capabilities ushers in a new era of efficiency and strategic advantage. It also propells the finance and banking sector towards sustained growth and success.
How financial services can invest in the future with predictive analytics
To stay ahead of these developments, banks and major financial institutions must prioritise investments in apt tech infrastructure. This may involve adopting cloud-based solutions, fostering internal expertise in NLP or chatbot development, and establishing collaborations with fintech startups. Moreover, emphasis should be placed on implementing stringent data governance and security measures to ensure compliance and safeguard against fraud.