Lately, we have heard so much about the benefits of generative AI for business adoption. It’s developing at an unprecedented pace, can do the work of hundreds in just a few seconds, and above all—it is cheap. But what does it really mean?
From the perspective of an individual, the cost is usually none, as most non-business use relies on free, limited access. But when corporate implementation comes into play, you have to include various factors in the final cost estimation to understand the cost of generative AI.
One thing is for sure—the development of generative AI systems has radically improved our access to artificial intelligence capabilities, whether on a private or business level. Open generative AI models available as a service have taken a huge chunk of work off companies’ shoulders, allowing them to dream big without wringing their hands over massive budgets.
In this article, we break down generative AI pricing, giving you tools that help with planning expenses and verifying received offers. Ready to dive into numbers?
Who spent the most on AI in 2024?
Before we jump into the intricacies of cost estimation, let’s take a quick look at the business expenses that generative AI has generated since its big boom. Banking and retail clearly take the lead based on various statistics, heavily investing in generative AI tools over the last two years—primarily for automating customer service. Other industries, particularly manufacturing, are stepping on their toes, increasing their generative AI budgets.
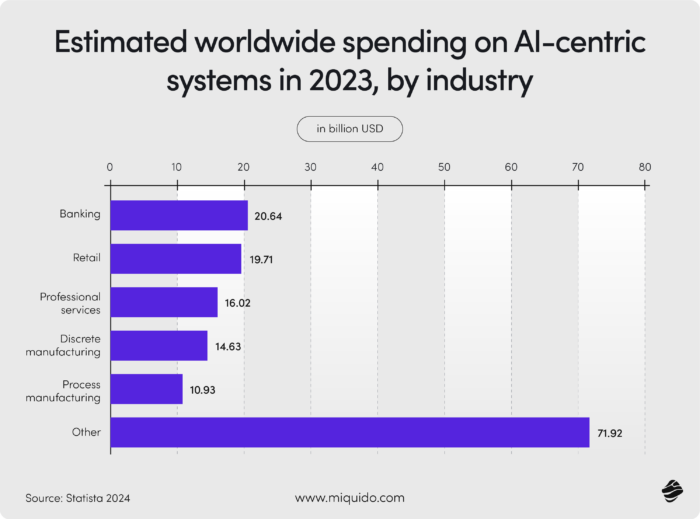
Estimates show that this trend will likely continue, with 60% predicted growth in generative AI budgets between 2024 and 2027. Companies across sectors seem to have noticed the potential of developing generative AI models and are ready to invest in them. The fact that, due to generative AI systems, these investments are just a fraction of what companies had to spend on artificial intelligence a few years ago contributes to this progress. But how does it look in reality? Which expenses do you really have to consider?
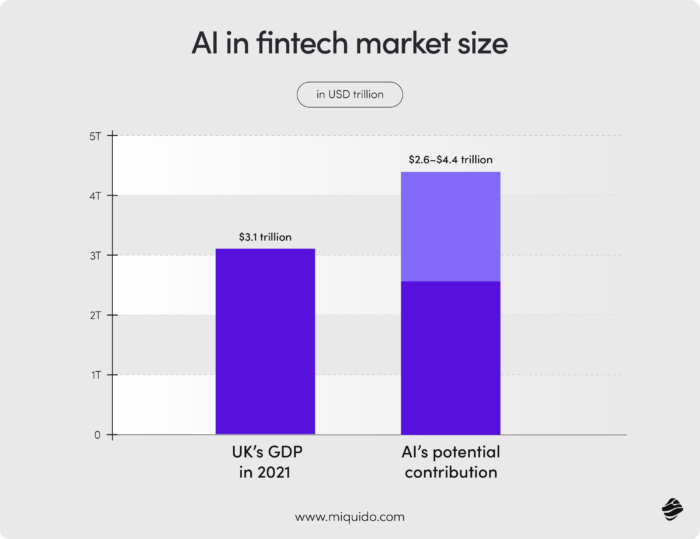
Is generative AI free to use?
The answer to this simple question is not as obvious as it might seem at first glance. Generative AI can be free, but for business users, it rarely is. The absolute majority of ChatGPT users worldwide restrict themselves to free, limited use. Among 200 million weekly users worldwide, only 10 million are paying subscribers.
Many people are not even aware that using these tools comes with expenses. But when it comes to corporate use, paying for the generative AI model is an integral element of the overall budget. How much does generative AI cost on average? When talking about generative AI tools, the choice of your model and its provider strongly determines the overall expenses.
The frequency of use is also crucial, as it impacts the number of utilized tokens, which are the billing foundation. With ready-made tools, you don’t need to train generative AI models, but ongoing expenses related to token use are inevitable.
There is not much public data on generative AI costs in companies, but by including the aspects below in your estimation, you can arrive at a realistic figure. If you are at sixes and sevens, worry not—let’s do the math together!
Factors influencing the cost of Generative AI solutions
Project spectrum
The purpose of your generative AI project will have a high impact on overall costs. Implementations that generative AI models are best equipped for will clearly require less involvement from your company, which means fewer expenses. These include chatbots and virtual assistants—features that are mature enough to integrate and start using immediately.
However, a lot depends on the volume of data acquisition and processing- even a simple chatbot can turn costly if handling a heavy data flow involving images, videos, etc. If you want to build a generative AI app such as an advanced personalization engine for e-commerce or dynamic ads, the budget will clearly expand. That is due to the computational power you will need to process input data at scale and the costs of scalable cloud computing resources for AI model inference.
If you combine GenAi with other technologies like machine learning or computer vision, you have to take additional costs into account. Take, for instance a module with
Expertise on board
Implementing GenAI systems requires a blend of IT expertise and domain-specific knowledge, leveraging existing AI models to tailor solutions for distinct industries.
Let’s compare two GenAi implementation scenarios to illustrate how your team can grow or shrink depending on your project. We will be comparing a conversational chatbot for banking customer service and a GenAI-supported drug discovery platform.
1st scenario: conversational chatbot for banking customer service
In the banking scenario, a conversational AI chatbot streamlines customer service, handling queries, complaints, and service navigation. By leveraging pre-trained natural language processing (NLP) models, the team adapts the system to understand banking-specific terminology and customer intent.
Key IT roles include:
- AI engineers, who adapt the chatbot for accurate and context-aware responses;
- software developers, who integrate the chatbot with core banking systems like customer databases and fraud detection platforms;
- security specialists, who ensure robust data protection measures.
- data scientists who monitor chatbot performance, analyzing trends to refine its capabilities.
- additional roles, such as customer experience designers, UX designers and compliance experts, ensure usability and regulatory alignment.
2nd scenario: GenAI-supported drug discovery platform
In contrast, the drug discovery scenario uses GenAI to analyze biological data and propose new molecular compounds, significantly accelerating research timelines. Instead of developing AI models from scratch, the team adapts existing generative AI frameworks to predict molecular interactions and identify promising compounds.
Here:
- GenAI researchers fine-tune models for drug-specific applications
- data engineers handle the vast and complex datasets involved
- machine learning engineers optimize the system’s predictive capabilities
- AI visualization specialists create user-friendly dashboards to interpret results.
Collaboration with domain experts, such as computational biologists and chemists, ensures scientific accuracy, while regulatory specialists align outputs with compliance standards.
Now, let’s count the potential costs of the core team, assuming to have two GenAI researchers, two data engineers, one machine learning engineer and one AI visualisation specialist on board. The combined half-year cost of this team falls between $365,000 and $455,000, based on average market salaries in 2024.
Sounds costly, doesn’t it? It doesn’t have to be. With our AI Kickstarter, you get all the key competencies for the fraction of traditional costs, excluding the expenses related to salaries.
Language model service provider and tokens use
The choice of the service provider has a mild impact on an overall costs, since the rates of the market leaders differ only slightly. The differences in expenses only start becoming significant with very intense use for the purpose of extensive systems.
What is crucial for your cost estimation, however, is the use of tokens. Tokens in large language models (LLMs) are the fundamental units of text, including words, parts of words, or characters, that the model processes to generate responses. LLM providers base their billing on token usage.
It’s important to differentiate between the prompt (user input) and the completion (model output), as the latter may cost twice as much as the former. The choice of the model among all oferred by one provider will also impact your overall costs. For instance, some models from OpenAI come with as little costs as $0.03 per 1000 tokens, but the most advanced models may be 4 times as expensive.
Provider | Model | Context | Input/1k Tokens | Output/1k Tokens | Per Call | Total |
---|---|---|---|---|---|---|
OpenAI | GPT-3.5 Turbo | 16k | $0.0005 | $0.0015 | $0.0020 | $0.20 |
OpenAI | GPT-4 Turbo | 128k | $0.01 | $0.03 | $0.0400 | $4.00 |
OpenAI | GPT-4o (omni) | 128k | $0.005 | $0.015 | $0.0200 | $2.00 |
OpenAI | GPT-4o mini | 128k | $0.00015 | $0.0006 | $0.0007 | $0.07 |
OpenAI | GPT-4 (8k) | 8k | $0.03 | $0.06 | $0.0900 | $9.00 |
OpenAI | GPT-4 (32k) | 32k | $0.06 | $0.12 | $0.1800 | $18.00 |
OpenAI | GPT-3.5 Turbo | 4k | $0.0015 | $0.002 | $0.0035 | $0.35 |
Mistral AI | Mixtral 8x7B | 32k | $0.0007 | $0.0007 | $0.0014 | $0.14 |
Mistral AI | Mistral Small | 32k | $0.002 | $0.006 | $0.0080 | $0.80 |
Mistral AI | Mistral Large | 32k | $0.008 | $0.024 | $0.0320 | $3.20 |
Meta | Llama 2 70b | 4k | $0.001 | $0.001 | $0.0020 | $0.20 |
Meta | Llama 3.1 405b | 128k | $0.003 | $0.005 | $0.0080 | $0.80 |
PaLM 2 | 8k | $0.002 | $0.002 | $0.0040 | $0.40 | |
Gemini 1.5 Flash | 1M | $0.0007 | $0.0021 | $0.0028 | $0.28 | |
Gemini 1.0 Pro | 32k | $0.0005 | $0.0015 | $0.0020 | $0.20 | |
Gemini 1.5 Pro | 1M | $0.007 | $0.021 | $0.0280 | $2.80 | |
DataBricks | DBRX | 32k | $0.00225 | $0.00675 | $0.0090 | $0.90 |
Anthropic | Claude Instant | 100k | $0.0008 | $0.0024 | $0.0032 | $0.32 |
Anthropic | Claude 2.1 | 200k | $0.008 | $0.024 | $0.0320 | $3.20 |
Anthropic | Claude 3 Haiku | 200k | $0.00025 | $0.00125 | $0.0015 | $0.15 |
Anthropic | Claude 3 Sonnet | 200k | $0.003 | $0.015 | $0.0180 | $1.80 |
Anthropic | Claude 3 Opus | 200k | $0.015 | $0.075 | $0.0900 | $9.00 |
Amazon | Titan Text – Lite | 4k | $0.00015 | $0.0002 | $0.0003 | $0.03 |
Amazon | Titan Text – Express | 8k | $0.0002 | $0.0006 | $0.0008 | $0.08 |
Industry
The question of industry also has key impact on overall costs of your generative AI solution. With the appearing legislative regulations, like the AI act, some industries ended up requiring more work and safety measures than others. The medical or governmental Ai systems, for example, require additional human oversight and compliance, making it more costly due to additional expertise on board.
Using external AI consulting support with a company experienced in your industry might be your middle ground, providing you expertise without recruitment and hiring costs. With our blend of extensive experience in artificial intelligence services and consulting background, we might be your perfect match.
Is it worth all this work? The numbers say it all. According to prognoses made by McKinsey, deploying generative AI in pharmaceutical and medical product industries could unlock value equal to 2.6 to 4.5 percent of annual revenues in these sectors. Banking could count on an additional $200 billion to $340 billion. So, in the end, it’s all a matter of balancing profit and loss.
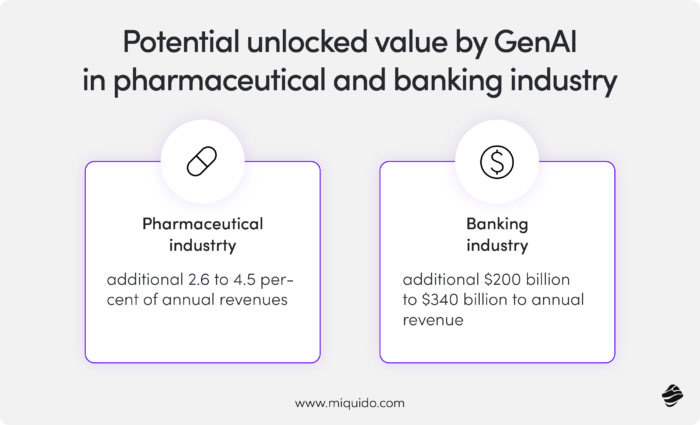
Key Generative AI implementation costs
As in any IT project, the overall cost of GenAi implementation depends on many factor. Analysing the aspects below step by step, you will be able to estimate realistic budget.
Cloud infrastructure
Cloud infrastructure is essential right from the beginning of implementing Generative AI. Whether you’re setting up a custom solution or using an existing platform (e.g., OpenAI, Google Cloud, Azure), cloud services provide the required computational power and scalability for hosting AI models, data, and applications.
While you need it to benefit the potential of GenAI, the cloud infrastructure comes as at a cost. You will have to count the monthly charges of cloud services into your budget. These costs will enhance as you scale your model.
Potential costs:
- Cloud compute (e.g., GPU/TPU usage): Depending on your workload, compute costs can vary from $0.10 to $5+ per hour.
- Storage: Data storage costs can range from $0.01 to $0.20 per GB per month.
API Integration
API integration is needed when leveraging pre-built Generative AI models through cloud providers or third-party vendors, which is most of current business scenarios. It’s implemented after cloud infrastructure is set up, and it connects your AI tool with customer-facing applications (websites, mobile apps, CRMs).
External APIs
The existing APIs provided by LLM service providers save you from the expense of building and maintaining your own AI models. However, you must account for the working hours your developers will need to establish this integration.
The better documented your API is, the less time it will take your team to fully integrate your solution. Pre-built libraries also help accelerate the entire process. On average, integrating a third-party API typically takes 1 to 3 weeks, depending on the project’s complexity.
Internal APIs
Although convenient, external APIs introduce privacy risks, particularly in regulated industries. Additionally, you are reliant on their functionality, which means potential disruptions if issues arise on the provider’s side.
Token costs will generally be a relatively minor expense unless you plan to deploy a tool serving users on a very large scale—yet, they still represent an additional cost. Internal APIs avoid these issues, but building them requires a significant investment.
Potential costs:
- API usage: For instance, OpenAI’s API pricing can range from $0.002 to $0.12 per 1,000 tokens, depending on the model used.
- Integration labor: Depending on the complexity, integration could cost anywhere from a few thousand dollars (for basic setups) to tens of thousands for enterprise-scale integrations.
Fine-tuning
Fine-tuning is applied after the initial setup and API integration. It’s most useful when the AI model needs to be adapted for specific business needs or domains (e.g., customer service, legal, or medical sectors). It ensures the generative AI model understands your particular terminology, context, and objectives.
Although not always necessary, it makes an integral part of a large chunk of GenAI implementations today. Particularly in the light of recent EU regulations, many companies treat fine-tuning as a preventive measure supporting compliance. At the same time, it can improve productivity – with domain-specific knowledge, the AI can better understand and interact with customers.
If you decide fine tuning is integral to your generative AI implementation, you should be conscious of the additonal costs it brings. The process of preparing datasets, training, and validating the model can take a significant amount of time, and time is money. Machine learning experts on board will be essential to carry out the fine-tuning succesfully. Plus, you should be aware of extra charges depending on your LLM provider.
Potential costs:
- Fine-tuning services: Fine-tuning can cost anywhere from $0.01 to $0.20 per 1,000 tokens, depending on the platform.
- Specialists: to succesfully carry out fine-tuning, you will need a Machine Learning specialists and data scientists on board.
- Training data preparation: Gathering and labeling data could cost $1,000 to $10,000+ depending on the amount and complexity.
Fine tuning is an expensive, but you can achieve similar results with well-implemented RAG. That’s one of the ways our AI Kickstarter framework cuts the costs. You can avoid the costs of hiring ML and data scientists while cutting development time. Your wallet will thank you later!
Retrieval-augmented generation (RAG)
RAG is implemented when you need the AI system to interact with external, dynamic knowledge bases. It’s often used in scenarios like customer support, where AI needs to pull real-time data or specific documents to answer questions accurately.
Aside from ensuring accuracy and thus, protecting your company’s reputation, RAG enhances the model’s ability to handle complex queries by combining generated responses with factual data retrieval. Also used for personalization purposes, it can access customer-specific data, enabling more personalized interactions.
Although it opens many new possibilities, companies don’t always decide to implement RAG due to needed integration with external databases or knowledge management systems. At the same time, searching and retrieving relevant information in real-time can increase the processing time, impacting the response time and cost.
Potential costs:
- Data storage and indexing: Costs for managing large data stores and implementing indexing systems could range from $0.05 to $0.15 per GB per month.
- API/Service calls: RAG integrations often require additional API calls to knowledge sources, with costs depending on usage (e.g., $0.001 to $0.10 per API call).
Enhancing accuracy vs. GenAI costs
Integrating with a Knowledge Base (KB)
To enhance your AI’s knowledge and accuracy, integrating with a KB is critical. This involves:
- Cloud Hosting: $500–$10,000/month (based on size and usage).
- Data Cleaning and Structuring: Domain experts and data scientists, $100–$250/hour.
- API Development: 4–8 weeks of engineering work, $50–$150/hour.
- Graph Database Tools: Licensing fees of $200–$2,000/month (e.g., Neo4j).
Adding a verification layer
Verification ensures output reliability through rule-based checks or human oversight.
- Development Costs: $5,000–$25,000 over 6–12 weeks.
- External Verification APIs: $0.01–$0.10 per call (scales with use).
- Model Training: $1,000–$30,000 (depends on dataset and complexity).
- Ongoing Maintenance: $2,000–$5,000/month for updates and support.
Introducing a fact-checking module
A fact-checking module validates outputs against trusted sources and enhances accuracy.
- Data Sources: $500–$10,000/year (subscription to APIs or databases).
- Model Development: $10,000–$100,000 for custom training.
- API Integration: $1,000–$5,000 (one-time cost).
- Human Moderators: $15–$50/hour for reviewing inconsistencies.
- Performance Monitoring: $500–$3,000/month for tools and dashboards.
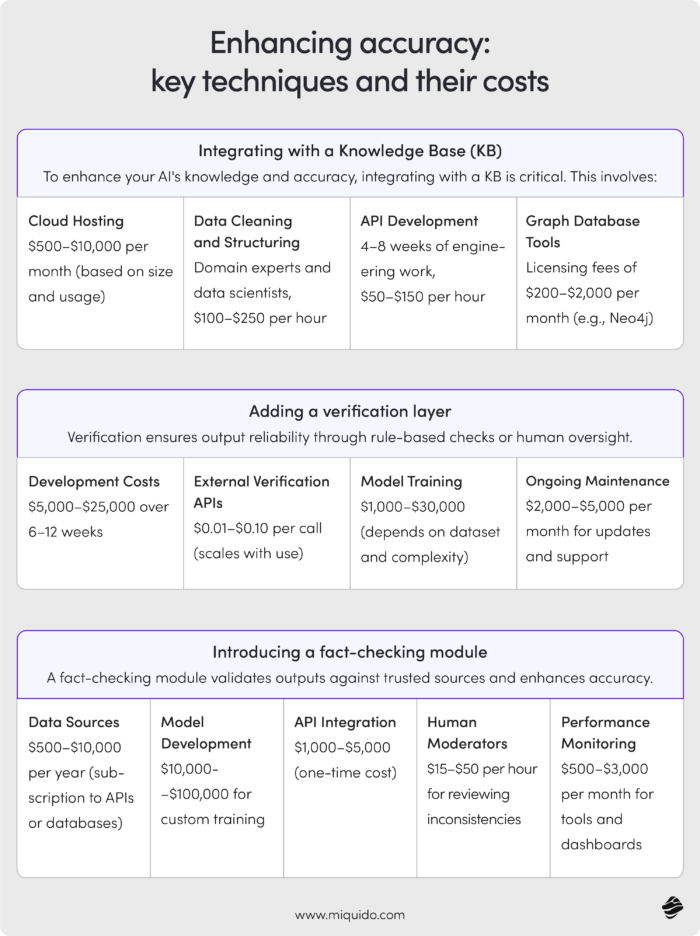
Implement GenAI at a fraction of costs
Based on the estimations above, the cost of generative AI system in a traditional model will likely reach at least $40,000. If you want to add elements that enhance model accuracy, you should expect additional expenses of $30,000–$40,000.
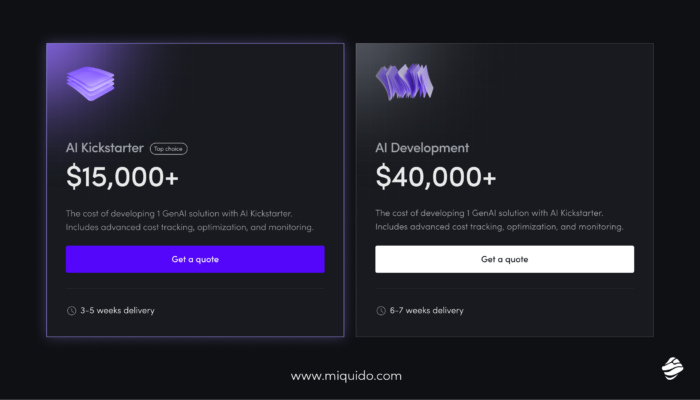
Knowing that expenses are often a barrier for companies considering GenAI implementation, we identified a solution that lowers the actual costs by an average of 65%. AI Kickstarter will allow you to push innovation forward faster and without having to gather an extensive budget on generative AI development.
Ready to unlock the double digit growth and develop a robust generative AI app with us? Let’s discuss your idea!