The transformative impact of artificial intelligence (AI) on the pharmaceutical industry is undeniable. The journey started in the 1990s when pharmaceutical companies experimented with basic AI models to formulate drugs. In the 2000s, machine and deep learning accelerated research and development, paving the way for the extensive use of AI in pharma.
Today, AI is changing every part of pharmaceutical industry, from research and development to supply chain and manufacturing. The result is end-to-end efficiency, enhancing patient health outcomes.
This article explores AI benefits, challenges, and insights for the pharmaceutical industry. We’ll discuss ways to implement this transformative technology into workflows and share real-life case studies of companies using AI to accelerate and optimize processes.
Industry stats: AI in the pharma industry
Before we examine how AI is used in pharma, let’s look at some interesting industry stats.
- AI in the pharmaceutical and medical-products industries is projected to create between $60 billion and $110 billion in annual value.
- AI could cut research development costs by $54 billion annually.
- AI-related patents in the pharma industry increased by 11% in 2024.
- Generative AI is a priority for 75% of pharma companies.
- The largest private investment in AI companies ($6.05 billion) was in medical and healthcare.
These findings show that the pharma sector is poised for an AI boom, with reduced costs and timelines driving huge profits.
Role of AI in Pharma
To appreciate its role in the industry, we must first understand what AI is. Artificial intelligence is a technology that enables machines to simulate human learning, problem-solving, decision-making, and creativity.
It does this through:
- Machine learning – algorithms that identify patterns and make predictions or decisions based on historical data.
- Deep learning – machine learning algorithms that process and analyze information.
- Generative AI – deep learning algorithms that create original content.
Check out our guides on ML vs Gen AI and Deep Learning vs Machine Learning to understand the differences between them.
These learning models are key in identifying new opportunities and improving operations in various sections of the pharmaceutical industry.
AI in drug discovery
An area with significant AI impact is research & development. Below is an illustration of the drug development process.
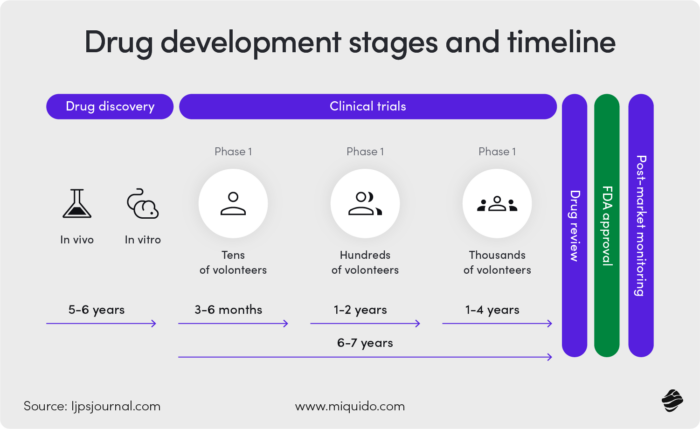
As you can see, the drug discovery and development phase is lengthy. It takes up to six years to find what causes disease progression and identify biomolecules that counter it.
AI accelerates this, extracting and analyzing information from various sources, including patent documents, scientific publications, conference abstracts, clinical trials, and public databases. Since it does this faster than humans, it can reduce discovery timelines by 25%.
That’s not all.
AI algorithms can predict the effectiveness of a new drug. Computer simulations identify chemical interactions that produce the best therapeutic effect, reducing the probability of adverse reactions before the clinical testing phase.
An accelerated drug discovery process means drug companies can create effective medicines in months rather than years.
AI in clinical trials
The time (and money) savings don’t end during the drug discovery and development phase.
Al also enables efficient and accurate candidate selection. Algorithms analyze patient records, identifying suitable participants based on specific criteria. The targeted recruitment ensures clinicians select patients most likely to benefit from the new therapy.
Predictive models trained on historical clinical data can be used to forecast outcomes such as drug efficacy. You can then use this insight to design successful drug trials.
A third application of AI in ongoing clinical trials is automation. Generative AI can automatically create artifacts like databases, study protocols, and clinical reports, giving clinicians more time to serve patients.
The results of targeted recruitment, predictive modeling, and automated processes are safer, faster, and more accurate clinical trials.
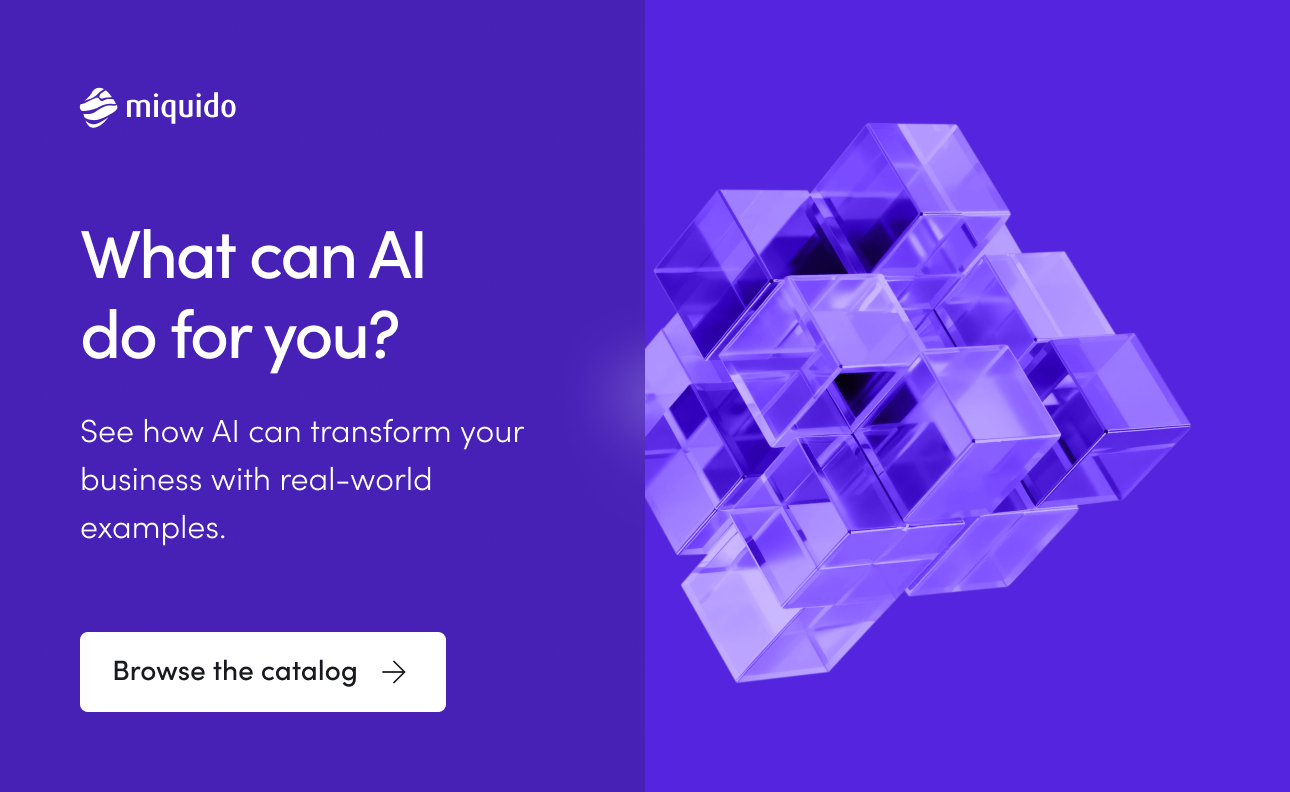
AI in supply chain management
Drug development and patient recruitment aren’t the only processes that benefit from AI’s predictive analytics. Predictive models can improve the availability of therapeutic drugs.
How is AI used in pharma supply management?
Two ways.
Manufacturing: AI models monitor equipment performance to support quality control. Predictive algorithms forecast equipment failures, minimizing disruptions. This real-time monitoring ensures regulatory compliance.
Inventory management: AI-integrated supply chains can predict spikes or dips in demand by analyzing historical data and market trends. That enables companies to optimize inventory management, reducing drug shortages and overstocking. It also improves the ability to mitigate potential bottlenecks by recommending alternative interventions.
The result is reduced waste, compliant systems, and efficient distribution networks.
Personalized medicine
AI in pharmaceuticals benefits patients as well as companies. Conventional medicine takes a generalist approach to care. Treatment focuses on the disease rather than the patient. Yet, symptoms, risk factors, and outcomes of the same disease manifest differently in various subgroups. For instance, heart attacks in women differ from men, and skin cancers look different in people of color.
The result is misdiagnoses and ineffective interventions for some patients. AI is changing this. Pharmaceutical companies will be able to improve patient outcomes with personalized treatment plans.
How?
AI algorithms can analyze genetic makeup, lifestyle factors, and medical histories to identify mutations and disease patterns. Pharma scientists can then develop medicines for patients with specific genetic profiles, reducing adverse reactions while improving treatment responses.
AI for drug repurposing
Sometimes, medical breakthroughs come from existing drugs rather than new ones. For example, Zidovudine was developed in 1964 to treat retroviral cancers. Twenty-three years later, it was repurposed as an anti-HIV therapy.
Drug repurposing identifies new uses for approved medicines. Researchers leverage existing drug profiles to explore their potential to treat different conditions, reducing time to market.
AI algorithms further accelerate the process of uncovering new patterns and associations between drugs, diseases, and biological pathways.
AI in pharmacovigilance
According to the World Health Organization, 1 in 30 patients suffer medication-related harm. Monitoring drug safety plays a key role in minimizing risk and adverse events.
AI in pharmacovigilance starts in the clinical trial phase. Continuously monitoring patient data helps detect early signs of adverse drug reactions. It doesn’t end there. AI identifies potential drug interactions by analyzing trial data, health forums, and patient records.
Drug safety monitoring is costly and time-consuming. AI automates and centralizes the collection and processing of adverse event reports, ensuring compliance with safety regulations.
Automation also reduces the workload associated with case documentation, accelerating the creation of scientific reports and lay summaries.
Benefits of AI in pharma
Application | Description | Benefits |
Drug discovery | Analyze datasets to identify potential drug candidates and predict their effectiveness. | Reduces the time and cost of drug development and improves the efficacy of new drugs. |
Clinical trials | Optimize trial design and participant recruitment. | Improves patient selection and automates artifact generation. |
Supply chain management | Monitor equipment performance, predict demands, and optimize inventory. | Reduces product waste and downtime, ensures regulatory compliance, and streamlines distribution networks. |
Personalized medicine | Analyze patient data to create customized treatment plans. | Improves treatment outcomes. |
Drug repurposing | Analyze existing drug profiles to find new uses. | Reduces time to market. |
Pharmacovigilance | Detect adverse reactions early. | Improve patient safety |
As you can see, you can apply AI to various sectors of the pharmaceutical industry. While we haven’t mentioned marketing and customer service, AI is revolutionizing those areas, too.
Current challenges of AI in pharma
With great potential comes considerable risk. Every industry adopting AI must grapple with the problems of AI implementation. Pharma companies have the added challenge of a strict regulatory environment governing data privacy and intellectual property.
Understanding AI risks allows you to develop effective policies to mitigate them. These are the factors you must consider.
1. Data privacy protection
Data privacy and security are major concerns in AI integration in pharma. Machine learning models train on large datasets, which include protected data like personally identifiable information, medical histories, and patents.
To guard against IP infringement and data privacy violations, you should train AI models on your intellectual property and adhere to data protection laws like the GDPR and HIPAA.
Your company’s security isn’t the only one you should worry about.
You’ll likely work with a custom healthcare software development partner to develop innovative AI solutions. Evaluate their security protocols to learn how they collect, use, and manage data. They should adhere to international standards for information security, GDPR, and HIPAA. Otherwise, you risk hefty fines and consumer trust.
2. Integration with existing system
A common misconception about AI is it’s easy to plug into existing systems and workflows. The reality is AI integration can be complex, especially with legacy systems.
Many legacy platforms are custom-built solutions that further complicate things because they don’t easily interface with newer technologies.
Modernizing and enhancing critical systems requires careful planning. Biotech and pharma businesses must assess their current infrastructure and processes to identify integration opportunities and challenges.
For instance, you should determine if you need a complete overhaul or modify the existing system incrementally.
3. Ethical dilemma
Ethics is a big issue in AI deployment. A 2024 KPMG survey found that 78% of consumers hold organizations that use AI responsible for its ethical development and usage.
One of the biggest concerns of using AI in the pharmaceutical industry is bias.
AI systems are only as good as the data they are trained on. Algorithms can be biased based on who builds them, how they’re developed, and how they’re used.
Historically, several population groups have been missing or misrepresented in clinical data. If executives make decisions about drug development and patient treatment based on this information, it could lead to fatal outcomes.
For AI to be ethical, it must be transparent and bias-free.
- Transparent: all stakeholders should understand how AI models make decisions.
- Bias-free: AI models must not perpetuate existing inequalities.
Since biases come from data collection, train algorithms on diverse data. You should also evaluate AI systems for outcome disparities across various groups and incorporate human oversight.
4. Compliance issues
The pharmaceutical industry is highly regulated. These companies must navigate multiple regulatory bodies to ensure compliance. There’s the Health Insurance Portability and Accountability Act (HIPPA) and the European Union’s General Data Protection Regulation (GDPR), to name a few.
These laws safeguard patient data by outlining the use and disclosure of said information. The primary principle across most data compliance regulations is notice/consent. You must notify and receive permission to use personal and medical data for purposes other than user requests. These include marketing and training machine learning algorithms.
Other ways to minimize regulatory and compliance issues include:
- Limiting data access to authorized or role-based users.
- Implementing multi-factor authentication
- Encrypting sensitive data
Recently, the European Union enacted the AI Act to address the risks of artificial intelligence and machine learning. It prohibits certain AI uses and defines obligations for providers of high-risk AI applications. Use the AI compliance checker to learn your obligations under the law.
5. Data preparation
Pharma companies have access to unprecedented volumes of data. The problem is machine learning models don’t distinguish between good and bad data, increasing the risk of AI hallucinations from incomplete or inaccurate data.
All that to say, data quality is a major concern.
The chart below shows the leading barriers to AI adoption in drug discovery.
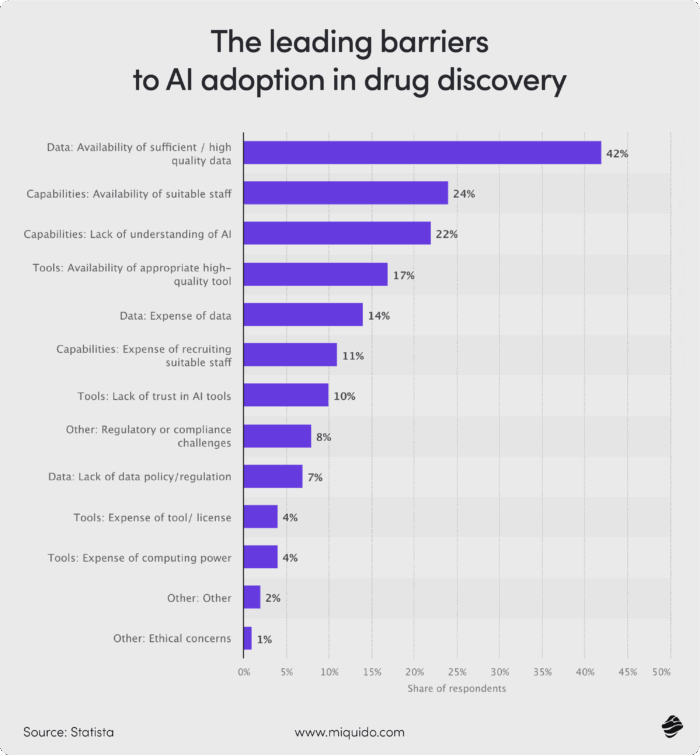
Quality data ranked first at 42%, followed by the availability of experienced employees.
Organizations must ensure their data is ready for sophisticated algorithms. Data preparation involves collecting, cleaning, and categorizing raw data, making it suitable for machine learning models. Failure to adequately prepare data will negatively impact AI projects.
You can also mitigate the risk of bad data with human oversight. Mechanisms like human-in-the-loop (HITL) or human-in-command (HIC) ensure accuracy and prevent undesirable consequences.
Despite fears that AI is stealing jobs, the opposite is true. Moreover, there aren’t enough experts to fill these vacancies. Fortunately, you don’t have to build an entire AI department in your organization. You can simply partner with top healthcare software companies to acquire AI solutions as you focus on your core business functions.
How to integrate AI in Pharma workflows
The question isn’t if you should integrate AI. Adoption trends show increased interest globally.
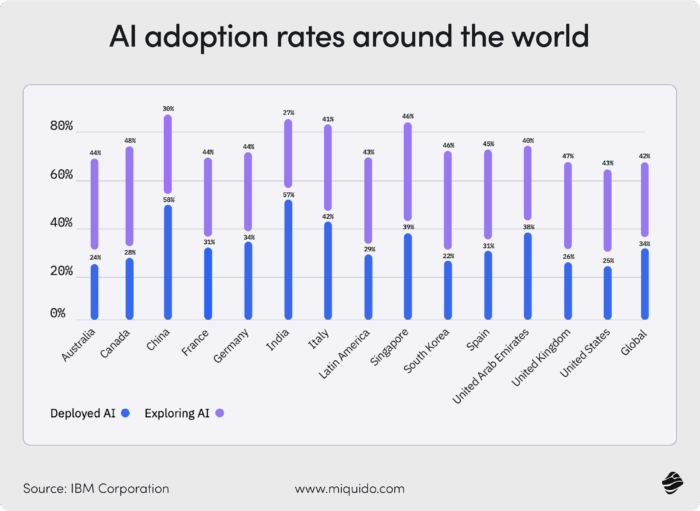
The question is how to integrate AI in pharmaceuticals. Hopefully, we’ve made the case by showing you various applications for the technology in the industry. However, you shouldn’t jump into AI integration without a plan.
Here’s our take on how best to tackle AI.
1. Start with clear objectives
The first step in implementing AI into pharma workflows is defining goals and objectives. You want to be clear about why you’re integrating and what you want to achieve. It could be as simple as increasing productivity by automating repetitive tasks or accelerating medical writing.
We recommend starting your AI journey with simple projects to minimize risk. Focus on content creation, medical writing, or chatbot implementation. They are low-risk and demonstrate value quickly.
For example, medical writing is time and resource-intensive. Generative AI simplifies and accelerates the process, allowing scientists to focus on other activities.
These areas also have clear and measurable metrics like cost, time savings, and quality. Early wins make further adoption of complex AI systems easier. Check out our guide to mobile apps in healthcare for insights on popular AI applications you can adopt for your starter project.
2. Data preparation and management
Data is the foundation of every AI system. You must feed your learning models clean, organized, and reliable data. The model will produce poor results if algorithms are tainted with irrelevant, incomplete, or biased data.
Data cleaning tools, like Integrate.io or Oracle Enterprise Data Quality, clean and organize data by removing duplicates, correcting errors, and structuring data into different formats.
Once cleaned and organized, segregate, encrypt, and store sensitive data in secured repositories, ensuring compliance with regulations.
3. Build cross-functional teams
Successful AI implementation relies on close collaboration of multidisciplinary experts with diverse ideas, perspectives, and expertise.
Cross-functional teams should consist of:
- Data scientists – train AI models
- Software engineers – integrate algorithms into pharma processes
- Subject matter experts – provide industry knowledge
- Business analysts – ensure business alignment
- UX/UI designers – create user-friendly interfaces
- Legal experts – ensure ethical and regulatory compliance
Bringing together specialists from data science, business operations, laboratories, etc., improves problem-solving, minimizes silos, and ensures alignment with business needs and objectives.
4. Design and plan effectively
Successful AI projects clearly define the problem they’re addressing. If you integrate AI because it’s trending, you’ll frustrate employees and waste resources.
Your implementation roadmap should follow these phases:
- Discovery – create cross-functional teams to uncover issues AI can solve.
- Planning – design data infrastructures and select appropriate learning models.
- Data preparation – collect, clean, and organize data that train AI models
- Development – build and train AI models, create user interfaces, and set up integration points.
- Testing – validate AI decisions
- Deployment – launch the AI solution.
We recommend using lean methodologies. The iterative development and continuous feedback provide real-time progress, allowing you to reprioritize requirements and mitigate risk. More on this later.
5. Focus on ethical and regulatory compliance
Ethics and accountability must guide your AI implementation. Regulatory and ethical compliance protects you from civil and criminal liability.
Create clear policies for data handling and algorithm transparency that align with regulatory and ethical requirements. Focus on building systems with safeguards and guardrails.
Safeguards include:
- Secure data handling practices like encryption and access controls
- RAG (retrieval augmented generated) architecture combines the general knowledge of large language models with business-specific or custom data.
Guardrails are rules or tools that detect AI hallucinations or AI biases. These include human oversight for high-risk tasks.
Following these practices ensures data integrity and fosters trust.
6. Leverage existing AI models
Building AI models from scratch isn’t necessary in most cases. Save time and money by leveraging pre-trained models from Google Cloud, Amazon Web Services, Microsoft Azure, and OpenAI, to name a few.
You can personalize existing models by implementing RAG architecture for business-specific outputs.
At Miquido, we’ve created an AI Kickstarter framework that uses your data and language learning models to build fast and safe apps.
7. Budgeting and measuring success
AI pricing varies depending on your industry and the complexity of the project. Estimates range between $50,000 to $500,000 for small to medium projects and $50,000 to over $5,000,000 for large-scale projects.
These costs cover development. There are also integration and maintenance costs.
To ensure a return on investment, you must define success with clear and measurable metrics.
Key ROI performance indicators are time savings, cost reductions, and operational efficiencies. For example, a positive ROI for a medical AI chatbot would be increased support and reduced labor costs. A predictive maintenance tool should deliver reduced costly downtimes in supply chains.
8. Team training and adoption
Resistance and a lack of understanding are significant barriers to AI adoption in pharma.
Leadership can set the right tone by communicating how AI aligns with company goals. For instance, you can share mobile app trends in healthcare that illustrate how natural language processing helps medical partners with diagnosis and treatment options.
Overcome skepticism and fear by starting with projects that can demonstrate quick wins and measurable results. Conduct team training to show that AI isn’t replacing jobs but enhancing them. When employees understand how AI works and the opportunities available, they will be open to a growth mindset.
9. Test and validate thoroughly
AI systems require thorough evaluation to prove accuracy, reliability, and compliance. Rigorous testing and validating are non-negotiable for compliance and ethical reasons.
There are several methods to validate AI algorithms.
- Functional testing: verifies the proper function of AI systems
- A/B testing: compares two versions of an AI model to determine which is more effective.
- Ground truth validation: compares AI results with known reference datasets
- Robustness testing: measures AI’s ability to handle dirty data or system failures
- Security and privacy testing: verifies AI’s resistance to cyber threats
- Bias testing: identifies data biases and AI decisions with negative ethical impacts.
- Compliance testing: verifies AI complies with specified regulations and standards.
You will need automatic and manual tests to identify potential issues. For instance, a function test of an AI chatbot requires human intervention to verify its ability to understand user queries and provide appropriate responses.
10. Iterative development approach
Iterative development is a great way to approach AI projects. Rather than linear development, it involves building and refining AI systems through a series of iterations. So, you would begin with a minimum viable product (MVP) and make improvements through testing and feedback.
An MVP is the most basic version of a product. It has enough features to attract early adopters and is used to validate the product’s concept.
Let’s say you want to create a drug interaction app for medical professionals. The MVP would be a simple search function where doctors enter two medications, and the output reveals the type of interaction.
See the example below.
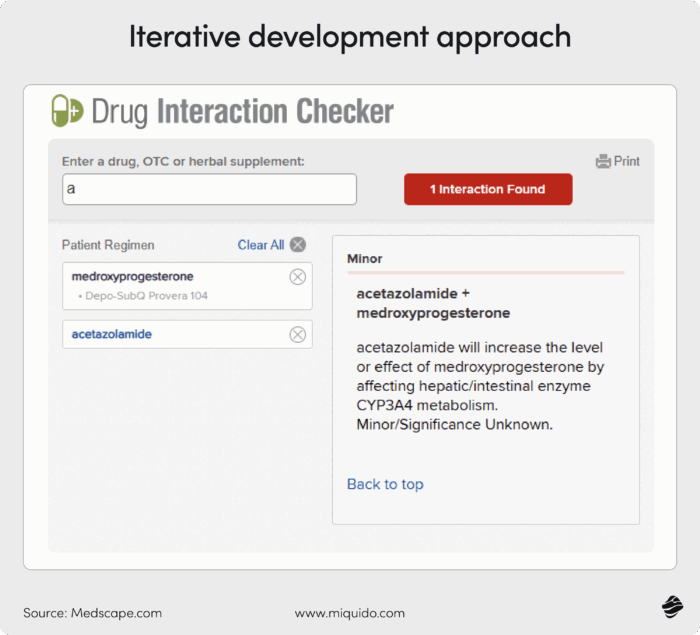
Later, you can add features like drug shortage alerts or patient-friendly communication techniques.
Sometimes our assumptions don’t align with market reality. MVPs allow pharma businesses to discover what resonates with users and create relevant products.
11. Partner with AI vendors
You don’t have to take your AI journey alone. Many pharmaceutical companies can’t develop machine learning systems in-house. Fortunately, you can outsource development, allowing you to meet demand and focus on core business functions.
Partnering with AI vendors is cheaper than creating and maintaining an internal team. You also access a global pool of specialized talent and cutting-edge technology.
Our process is end-to-end. We start with ideation, helping you find use cases for AI implementation in your organization. From there, we guide you in defining project scope and budget considerations. We remain with you post-development, maximizing the value of your investment with continuous improvements.
AI in Pharma case studies
Now, let’s look at how real-life pharma companies leverage AI technologies.
Johnson & Johnson
Johnson & Johnson uses artificial intelligence in several ways.
Drug discovery: applying AI to de-identified medical datasets like electronic health records, lab results, and genetic sequencing data to understand what drives specific diseases. Once disease targets are identified, AI is leveraged to discover, design, and optimize molecules to combat the disease.
Patient recruitment: applying AI and machine learning algorithms to de-identified datasets to locate research sites with patients that could benefit from trial medicines. Advanced analytics also help identify clinical locations with diverse patients.
Personalized medicine: applying AI to genomic and diagnostic tests to create tailored treatments. Johnson & Johnson is developing an AI-powered biomarker test to detect FGFR-positive tumors in digitized images of biopsies.
Supply chain: applying AI to demand fluctuations and supplier performance to prioritize hospitals, pharmacies, and clinics with high demand.
AstraZeneca
AstraZeneca uses AI for drug discovery. The global biopharmaceutical company discovered two drug candidates for chronic kidney disease and three targets for idiopathic pulmonary fibrosis.
The discoveries were enabled by integrating AstraZeneca’s data with an AI drug discovery platform and Knowledge Graph.
These technologies rapidly contextualize data from scientific literature, patents, genetics, and chemical and clinical trial reports. They helped scientists understand underlying disease mechanisms and identify novel drug targets.
Pfizer
Pfizer used AI in pharmacovigilance and drug safety monitoring during the COVID-19 pandemic. The unprecedented volume of vaccinations meant billions of cases of people sharing side effects related to the vaccine.
The global pharmaceutical company leveraged AI to automatically process and analyze adverse events from its multi-lingual reporting portal (COVAES), automated conversation AI solution (Agent LEO), medical journals, and the European Medicines Agency.
Pfizer reduced case processing cycles, giving scientists and safety personnel timely access to critical information.
In closing
Artificial intelligence improves decision-making, innovation, and operations in the pharmaceutical industry. Companies are poised to reap huge profits resulting from faster breakthroughs and enhanced efficiencies.
This article discussed key areas where AI is revolutionizing how companies operate.
So, how is AI used in pharma? Let’s recap.
- To accelerate research
- To predict drug efficacy
- To optimize clinical trial recruitment and monitoring
- To personalize medical therapies
- To automate processes
Note that the technology alone doesn’t guarantee success. You must understand how to use it and the challenges of integrating AI systems into existing workflows. That’s where an experienced partner like Miquido comes in.