With the economy remaining unstable since the 2008 crash, financial institutions have been strengthening security measures to safeguard their financial stability. The COVID-19 pandemic highlighted the critical importance of robust identity verification and KYC (Know Your Customer) processes.
The limitations posed by lockdowns exposed vulnerabilities in online-only tools, as banks struggled to thoroughly verify the identities of new customers. These gaps facilitated fraudulent activities: credit card fraud increased by nearly one-third, and identity theft surged by 53% between 2019 and 2020 (the outbreak year).
KYC, a framework applied by institutions for decades, has not stopped financial crime and identity theft from growing despite the hopes. However, the AI-powered procedures could turn the tide of events, empowering companies and their customers. Could AI make it impossible for the fraudsters, mafias or terrorist organisations to launder money? Let’s take a closer look at generative AI in banking and finance, specifically for KYC processes.
What is KYC (Know Your Customer)?
KYC (Know Your Customer) is a process used by financial services institutions to verify the identity of their clients and assess potential risks, such as fraud or money laundering. It prevents banks from storing and processing money from illegal activities and allows them identify patterns potentially linked to criminal activities
Remember Tom Ripley and its falsified identity granting him income for years? Before the KYC framework, this was a realistic scenario. Everything started changing in the seventies, with the formation of the Bank Secrecy Act (BSA). The birth of Financial Action Task Force (FATF) in 1989 underlined the need for international efforts against financial fraud, pointing out a set of values that today’s global regulations rely on.
However, it was not until the beginning of the 21st century that the pressures for robust KYC frameworks began intensifying. The 9/11 terrorist attacks were an international impulse to strenghten and unify these strategies. Just as it enforced new security measures in airports that stayed in power until today, they also forced banks to monitor customer activity to a much greater extent.
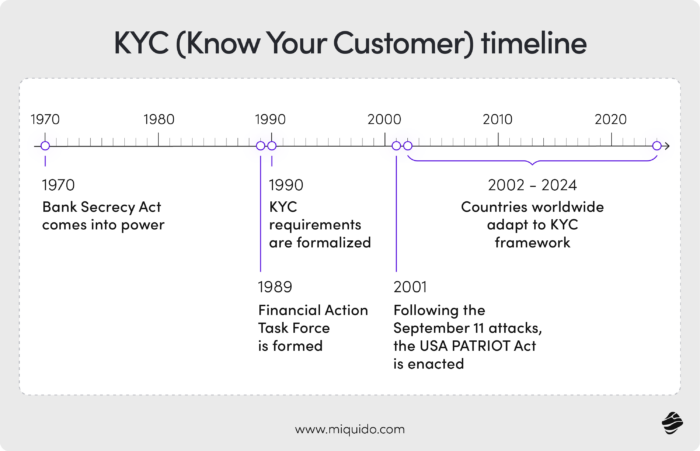
Key components of the KYC process
Although the implementation of KYC frameworks differs accross the globe, its core elements remain universal. They include:
- Customer Identification: Collecting and validating personal information, such as name, address, and date of birth, often with government-issued ID verification.
- Customer Due Diligence (CDD): The process of assessing customer risk profiles to determine the level of scrutiny required. This may include checking criminal records or financial history.
- Enhanced Due Diligence (EDD): For higher-risk customers, EDD requires deeper investigations into the source of funds, financial activity, and potential political exposure.
- Ongoing Monitoring: Constantly monitoring transactions to detect any suspicious activities that may indicate illegal behavior or risk to the financial system.
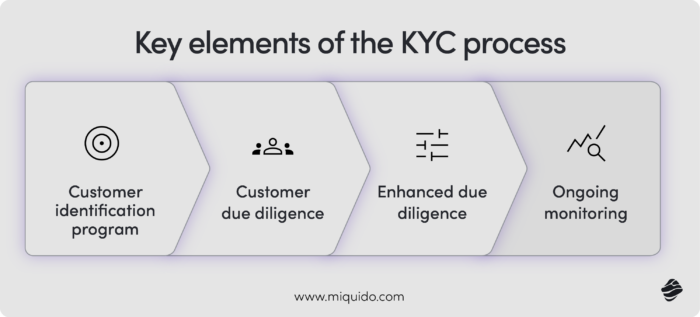
AI for KYC: how can it revolutionize KYC processes?
KYC proceses, like due diligence and monitoring, are all about detecting patterns. Fradulent activity rarely shows itself as an isolated act – it takes a bunch of data to identify inconsistencies.
Imagine a shell company set up as a fake import-export business, claiming to trade luxury goods. On paper, the transactions look legitimate: invoices match market prices, and payments flow through reputable banks. However, over time, subtle inconsistencies emerge—such as payments originating from high-risk jurisdictions, unusually repetitive invoice amounts, or discrepancies in shipping records.
These patterns, when observed across multiple transactions and compared to broader data, can reveal a classic case of money laundering. And it is literally impossible to do it manually with the amount of transactions passing through the bank day by day. AI is a perfect tool for it, enabling the institutions stay in line with KYC principles.
Where can it help?
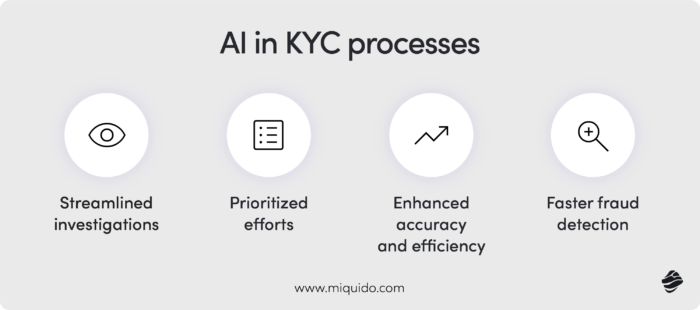
Streamlining investigations
Think about going through the financial records of a company, table by table—the sheer volume of numbers to process and scrutinize. Or imagine combing through endless global sanction lists to confirm a customer’s legitimacy. Even the most detail-oriented individuals are bound to make mistakes sooner or later.
AI alleviates this burden by automating repetitive tasks, enabling automated document verification, cross-checking information against databases, and scanning and validating IDs without manual intervention.
Prioritizing efforts
The challenge of detecing fraudulent patterns is already an obstacle, but you also have to know where to start from. Otherwise, identifying potential criminals it is like searching needle in a haystack. Effective prioritisation can push this forward, allowing you to filter the customers that do not need screening before diving deep into their data, wasting resources and computational power.
AI tools can identify high-risk customers by analyzing transaction patterns and applying predictive models to flag suspicious activities. This way, you save human resources necessary for more complex cases instead of overcharging them with manual tasks.
Enhancing accuracy and efficiency
Before the AI systems started being widely adopted in banking, the institutions strongly depended on user reporting. It usually took a card missing in the wallet or the emptied account for the customer to report crime and allow the bank to take appropriate steps. When it came to fradulent activity, like money laundering, the criminal activity could easily hide in the river of transactions the security teams would never have time to tackle on.
AI systems, on the other hand, can analyze massive datasets in real-time to identify deviations in customer behavior that may indicate fraud, money laundering, or other financial crimes. Instead of letting the issues escalate and the fraudulent organizations estabilish themselves and spread their activity internationally.
Is KYC the same globally?
KYC is part of a broader framework, such as Anti-Money Laundering (AML) and Counter-Terrorism Financing (CTF), that many countries enforce through specific laws. KYC practices share core principles worldwide but vary by region due to differing regulatory frameworks:
- United States: KYC is governed by the BSA, Patriot Act (2001), and FATF recommendations. Institutions focus on identity verification, transaction monitoring, and reporting suspicious activities.
- European Union: The Anti-Money Laundering Directives (AMLD) regulate KYC, requiring stricter customer due diligence, including beneficial ownership disclosures and cross-border cooperation.
- Asia: KYC standards vary significantly across countries. While regions like Singapore and Hong Kong have advanced frameworks aligned with FATF, developing nations may have less stringent implementations.
- Australia: Governed by the Anti-Money Laundering and Counter-Terrorism Financing Act (AML/CTF Act), Australia’s KYC rules are closely aligned with FATF standards, requiring detailed customer identification and ongoing monitoring.
- Latin America:Various Latin American countries, like Brazil, Mexico, or Argentina, are on a path to ensure KYC compliance aligned with FATF measures. However, the level of KYC adoption strongly differs accross the region, correlated with the economic growth.
- Africa:South Africa leads the continent with advanced KYC regulations aligned with FATF and its Financial Intelligence Centre Act (FICA), emphasizing identity verification and reporting suspicious activities. The compliance in other African countries is expanding but still in a
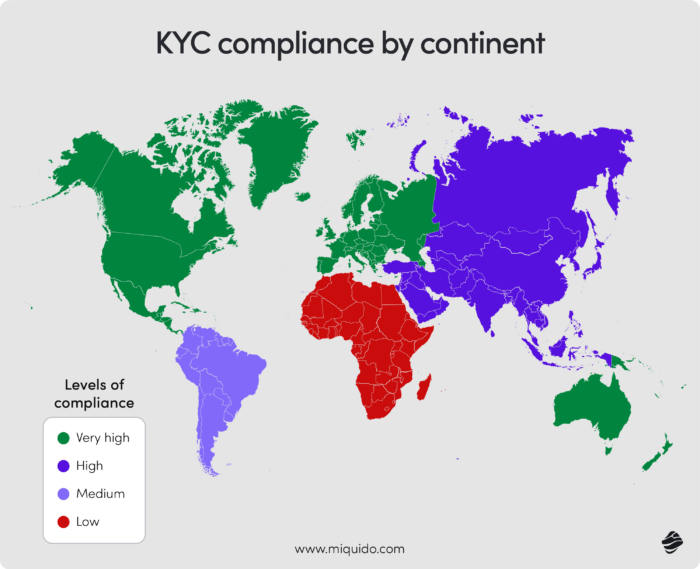
Adopting AI for KYC: challenges
Preventing data breaches
AI in KYC relies on sensitive data to analyze customer transactions and profiles, making it a potential target for cyberattacks. The adoption of AI increases security capabilities but also attracts cybercriminals attempting to exploit the constant flow of data feeding the algorithms. Strengthening cybersecurity is critical, especially considering the 75% rise in cyberattacks recorded in Q3 of 2024 compared to the same period in 2023.
How to handle it?
- Strengthen cybersecurity measures: Use advanced encryption methods and intrusion detection systems to protect sensitive data.
- Implement zero trust architecture: Minimize access to sensitive data by applying strict user verification for every access point.
- Regularly update security protocols: Ensure that your AI systems are protected against emerging threats through regular updates and patches.
Adhering to GDPR and other regional data protection laws
KYC procedures must align with stringent data protection regulations like GDPR. These regulations mandate adherence to principles such as data retention limits, user rights to data deletion, secure cross-border data transfers, and maintaining transparency about how personal data is processed.
How to handle it?
- Automate compliance monitoring: Use AI tools to ensure KYC processes meet regional and global regulatory requirements.
- Maintain transparent documentation: Keep comprehensive records of how AI systems handle data to meet audit requirements.
- Collaborate with legal experts: Engage professionals to align your data practices with applicable laws in different regions.
While countries share core data protection principles, specific regulations can vary widely. For instance, U.S. sector-specific regulations like HIPAA and GLBA differ from the EU’s GDPR, especially regarding retention policies and transparency. Globally, regulations in countries like Brazil and South Africa often mirror GDPR but lack consistent enforcement.
How to handle it?
- Standardize around GDPR compliance: Treat GDPR as a baseline to ensure alignment with the strictest standards.
- Adapt to local laws: Customize your KYC processes to accommodate unique regional requirements for global operations.
- Leverage cross-border data transfer solutions: Adopt secure solutions, such as data localization or privacy-enhancing technologies, to comply with transfer laws.
Improving data quality and reducing bias in AI models
Outdated, inconsistent, or biased data can lead to false positives or missed fraud risks, undermining KYC effectiveness. Bias in AI models can unintentionally reinforce discriminatory practices, causing the systems to either overreact to minor risks or overlook critical ones.
How to handle it?
- Establish data governance frameworks: Implement centralized systems to ensure high-quality, up-to-date data.
- Audit AI models regularly: Perform regular checks to identify and mitigate potential biases.
- Diverse training data: Use datasets that are representative of all customer demographics to prevent reinforcing existing biases.
- Implement feedback loops: Continuously improve AI models by incorporating insights from real-world use cases.
Ensuring compliance in AI-driven KYC processes
Data accuracy and quality
Companies must ensure that the data used to train and operate AI systems for KYC is accurate, complete, and up-to-date. Incorrect or incomplete data can lead to false positives or negatives, which could hinder compliance.
How to achieve it:
- Implement robust data validation processes to ensure incoming data is accurate and formatted correctly.
- Regularly clean and update datasets to remove outdated or irrelevant information.
- Use automated tools to cross-check data against trusted third-party sources such as credit bureaus or government records.
Ongoing monitoring and screening
AI systems must be used to perform continuous customer due diligence (CDD). This includes identifying suspicious transactions, monitoring for unusual behavior, and cross-checking with updated sanctions lists, politically exposed persons (PEPs), and adverse media databases.
How to achieve it:
- Integrate AI models with real-time monitoring systems to continuously scan for suspicious activity.
- Update AI algorithms frequently with new information from sanctions lists, PEP databases, and adverse media reports.
- Set up alert thresholds to ensure quick detection of anomalous patterns in customer behavior.
Auditability and explainability
AML regulations require companies to maintain transparent and explainable AI models. The decisions made by AI systems (e.g., why a customer was flagged as high risk) must be traceable and understandable to both regulators and internal compliance teams.
How to achieve it:
- Use interpretable AI models, such as decision trees or rule-based systems, where possible.
- Maintain detailed logs of AI decisions to provide traceability for audits.
- Implement user-friendly dashboards that compliance teams can use to understand and validate AI-driven decisions.
Independent validation
AI tools used in KYC must undergo periodic testing and validation to ensure accuracy and effectiveness in identifying money laundering risks. External audits may also be required in some jurisdictions.
How to achieve it:
- Schedule regular internal testing of AI models for accuracy and compliance with AML requirements.
- Engage external auditors to evaluate the system’s performance and identify potential biases or risks.
- Perform stress testing to assess how the AI system handles edge cases and high-risk scenarios.
Human oversight and accountability
Regulatory frameworks emphasize the need for human intervention in AI-driven decisions. Companies are responsible for ensuring that compliance officers review and act on AI-generated alerts, especially for high-risk cases.
How to achieve it:
- Assign compliance officers to review and validate AI-generated alerts and flagged cases.
- Train staff to interpret AI findings and make informed decisions.
- Establish clear protocols for escalating high-risk cases to human supervisors.
Reporting requirements
Companies must ensure AI tools facilitate Suspicious Activity Reporting (SAR) as per AML obligations. These systems must flag and report anomalies promptly to the relevant authorities.
How to achieve it:
- Configure AI systems to generate and organize reports in formats compliant with local regulatory requirements.
- Automate the generation of SARs to reduce delays in reporting suspicious activity.
- Ensure the AI system tracks and logs all flagged activities to facilitate regulatory report
Adapting to evolving threats
AI systems must be regularly updated to address new methods of money laundering and comply with emerging regulations. Companies must remain vigilant in adapting their AI capabilities to tackle evolving threats.
Today’s financial fraud landscape and the importance of KYC
Over the last few years, financial crime has continued to escalate, displaying concering trends. In 2024, 69% of global executives and risk professionals anticipate a rise in financial crime risks over the next 12 months, driven primarily by cybersecurity challenges and data breaches.
This growing threat underscores the urgent need for stronger regulatory compliance and enhanced risk assessment frameworks. Within the European Union, new regulations aim to provide clearer guidelines to address these concerns.
The KYC process is a cornerstone of these efforts, designed to reduce risks such as money laundering and other forms of financial crime. By streamlining identity verification and embedding AI-driven solutions into the KYC framework, financial institutions can significantly enhance their ability to detect and prevent suspicious activities.
With increased security come other benefits. Take HSBC, which is already succesfully using AI KYC solution, delegating it the tasks linked to research and due dilligence. As a result, their onboarding times reduced by more than 50%. Mastercard also reported improved customer experiences and higher compliance rates as a result of integrating artificial intelligence into their KYC process.
Adapt AI for KYC
Have you thought about including AI in the KYC process? With our AI and machine learning services backed by years of experience in banking and finance, you can streamline these operations while ensuring KYC compliance.