AI applications are essential tools for improving business operations. However, the app development process can be a cost-intensive venture. Depending on the project’s needs, scope, and scale, the overall cost of running an AI app development project can be very steep.
In this article, we’ll provide a comprehensive breakdown of the costs involved in AI projects, from initial concept to deployment and beyond. You’ll also learn the strategic importance of investing in AI.
So, let’s first examine the value of AI in business to see how different types of AI applications serve various business functions.
Tailoring AI Solutions to Business Needs
As of 2023, EY (Ernst and Young) revealed that 90% of respondents were open to exploring AI platforms like ChatGPT, Bing Chat, and OpenAI. The survey also discovered that 80% of tech executives intend to increase their investment in AI.
These stats reveal that companies are starting to recognize AI as an essential tool for maximizing business operations.
Undoubtedly, AI applications have become vital tools for improving workflow, business processes, and even collaborations. Below are business functions that AI can enhance.
- Marketing and sales – Businesses can use applications that provide AI-based personalization for shoppers to recommend unique products based on user behavior. This will likely generate more revenue and improve customer experience.
- Lead qualification – AI lead qualification based on pre-defined criteria can help businesses target customers who are more likely to convert. This enhances the efficiency of your marketing and sales processes.
- Market analysis – AI can help businesses analyze market trends and predict future demands, helping them maximize growth opportunities more efficiently.
- Human resources – Platforms like Zoho and Bamboo HR are starting to use AI to automate certain recruitment and talent acquisition activities, such as screening resumes to identify qualified candidates, scheduling interviews, and onboarding new hires.
- Accounting and finance – AI apps like Freshbooks or SAP Finance AI can help maintain proper financial accounting records. They can also analyze financial data for fraud detection or risk identification purposes.
There are far more AI and generative AI use cases than we’ve highlighted above. For instance, we have cybersecurity AI applications, inventory management, product conceptualization, and AI-based virtual assistants.
Companies can either go for off-the-shelf products or choose custom artificial intelligence solutions, depending on their specific organizational needs. If you’re torn between which to go for, assessing the cost implication for both can help you decide. So let’s do that.
Custom AI Solutions vs Off-The-Shelf Solutions
Custom AI solutions are tailored to specific needs and business processes, so they are likely to offer greater control and flexibility. However, custom applications may have significantly higher upfront costs due to the development needs, data, and infrastructure needed to keep the app running.
Custom applications also tend to take longer to build. More intensive iteration and testing will be needed to ensure the application is functional and its features effectively serve your business needs.
Custom applications may also require an in-house app development team with AI expertise. This may be more expensive to maintain over a long period. That’s why most businesses prefer partnering with a Generative AI development company like Miquido. This would allow you to build a custom AI app that meets your needs at a more reasonable budget.
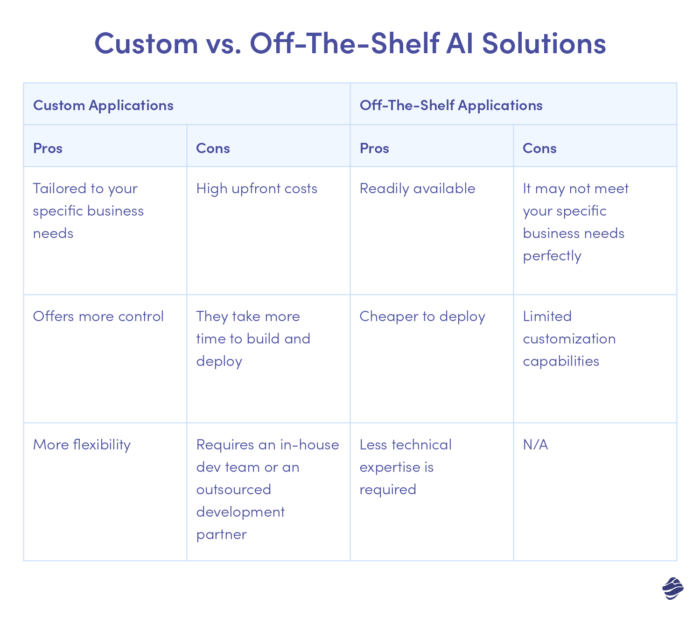
Off-the-shelf AI solutions are readily available and useful for broader business needs. This means they usually have lower initial costs since you don’t build the app from scratch. That also implies that deployment would be faster.
Moreover, off-the-shelf AI applications demand less technical expertise as they typically come with vendor support. This eliminates the need for an in-house team for AI app development.
Unfortunately, off-the-shelf solutions may not perfectly meet your specific business needs and have limited customization capabilities.
The choice between off-the-shelf solutions and custom solutions would majorly depend on two things: budget and scalability.
If you have enough funding and want a flexible solution that can adapt to your business as your operations grow, a custom solution is the best bet. However, if you need a budget-friendly solution that doesn’t require overly specialized functions, go for an off-the-shelf AI application.
AI App Development Cost: The Breakdown
Developing an AI app involves various phases, from initial research and development to integration with existing systems and ongoing maintenance. Each stage has associated costs.
Here’s a typical overview of what over 100 app development companies spend in each phase, according to a survey by Clutch:
- Initial Research and Planning
The initial research and planning phase includes market research, feature planning, wireframing, and basic prototyping.
This phase also entails significant data acquisition for training your AI model. Data acquisition can be quite expensive when working with large data sets. The specific costs will vary based on data sources, licensing fees, and other requirements.
Depending on the scope of your initial research or app design, you may spend between $5,000 and $50,000.
- Development and Integration with Existing Systems
Costs incurred during the development phase will depend on how complex your app model is, the computing power, and the level of expertise you need. The development stage would cost approximately $5,000-$10,000.
In the integration phase, you’ll integrate the app with your existing IT infrastructure, which may incur additional AI app development costs—especially when modifications are needed.
Additionally, if your app interacts with data from external devices or sensors, it might require integration with a Bluetooth Low-Energy (BLE) protocol. This integration process typically adds an estimated cost of $8,000 to $10,000 or more to the overall development budget.
Next, you’ll deploy your app. Depending on the app’s process and storage needs, you might need to use cloud infrastructure here. Costs may vary based on usage and chosen provider, but a budget of $500-$700 monthly should suffice if you’re working with top providers like Google, AWS, or Azure.
- Ongoing maintenance
Ongoing maintenance costs include bug fixes, improving functionalities, and implementing necessary updates.
Your app also needs data monitoring and retraining as it interacts with users and generates data. The costs for these depend on the frequency and complexity of your updates.
However, the accepted industry average for software maintenance is about 15-20% of its actual development cost.
How Much Does it Cost to Develop an AI?
The total cost of developing simple apps varies from what you’d spend on complex AI-based apps. This is often due to factors like the types of data used and the complexity of features or functionalities.
1. Internal knowledge bases.
Simple internal knowledge bases are typically centralized libraries with information for your employees. Some of these solutions include knowledge bots, automated IT support, customer service, and HR help desks based on GenAI. These types of applications usually cost between $5,000 and $8,000.
2. Complex Gen-AI based applications.
Complex Gen-AI applications often involve intricate algorithms, specialized features, and extensive data processing. As a result, the cost would be approximately twice as much as that of simple AI app platforms.
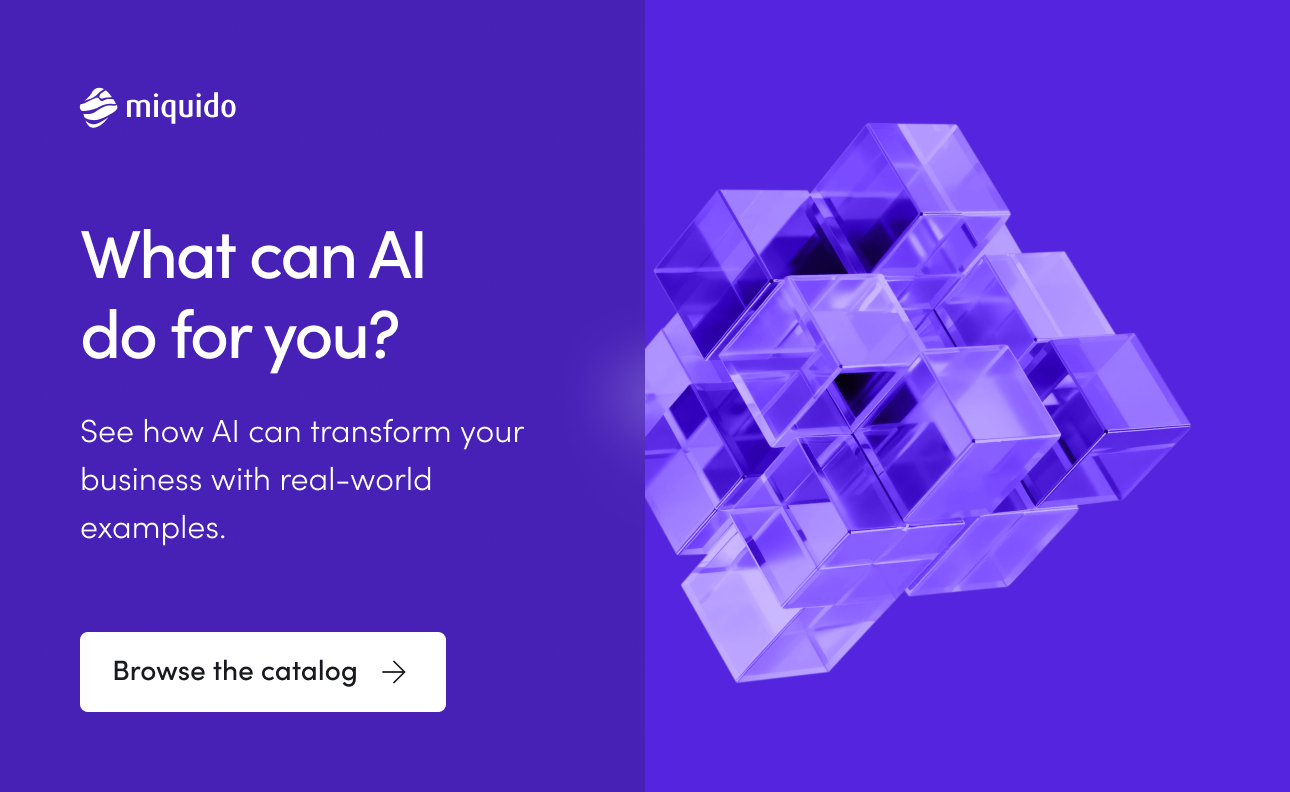
How to Budget for AI Projects
Accurately budgeting for AI projects is crucial to avoid cost overruns. Here’s how you can effectively budget for your AI app project:
- Define the project scope and goals.
Clearly define the problem(s) your AI application will solve. Highlight desired functionality and expected outcomes.
Then, break down the project into smaller phases, indicating clear deliverables and milestones so that you can have a more precise cost estimation.
A well-defined project scope prevents scope creep and unexpected expenses.
- Cost forecasting.
Next, develop a comprehensive cost breakdown structure (CBS) that identifies all potential costs associated with your project.
Include direct costs like salaries, hardware, data acquisition, vendor fees, and indirect costs like planning costs, training and documentation, maintenance, etc.
Potential risks or challenges should also be considered, as well as prevention strategies.
This is where working with an experienced AI app development company comes in handy. These companies have historical data that can help to forecast development costs more accurately.
- Choose a cost estimation technique.
Adopt cost estimation techniques like industry benchmarks, bottom-up estimation, or parametric estimation to determine a realistic cost estimate for the various elements in your CBS.
Industry benchmarks would typically be based on researching similar AI projects to ascertain the average development cost. Other techniques include:
- Parametric estimation – uses historical data from past projects to estimate costs for similar tasks in your current project.
- Bottom-up estimation – sums the approximate cost for individual tasks to determine the cost of the entire project.
We recommend creating a flexible budget with a contingency buffer (say, 10 – 20%) for unexpected challenges, delays, or changes in the project scope.
Importance of Data in AI Development
Data fuels the learning process of AI models. It’s through data that these models can identify patterns, make predictions, and perform other tasks. For instance, AI solutions like Netflix’s recommendation engine and Google’s Gemini AI thrive on high-quality data.
Data is also used to assess the performance of AI models after they have been trained. This primarily helps to identify and resolve issues with the machine learning process or relevant algorithms.
Three major processes are involved in using Data for AI— acquisition, analysis, and preparation. Let’s explore them and see the costs associated with each:
- Data acquisition
This involves sourcing the data used to train your AI model. To acquire data for training your AI models, you can use vendors who sell pre-existing datasets. You can also gather your own data using specialized data acquisition systems.
Using pre-existing datasets would require paying vendors for licensing or usage. This can be pretty expensive and would vary based on the data’s size, quality, or exclusivity.
If you wish to collect your own data, you’ll also need to factor in the costs of data acquisition (DAQ) systems. Low-end DAQ systems cost about $200-$500 per channel, mid-end systems cost roughly $500-100 per channel, and high-end DAQ systems range from $1,000-$2,000 per channel.
Finally, some data types may require human labeling/annotation (for instance, images used for object recognition). Annotation costs vary based on the data size and complexity.
- Data analysis
It involves assessing the structure and content of your data to understand its basic properties.
Here, you’ll calculate summary statistics, visualize data distributions, and identify potential outliers or missing values. The aim is to inform your data preparation steps by highlighting areas that need cleaning or transformation.
Outsourcing data analysis services may cost over $2500 monthly. Service providers typically charge for licenses and the number of hours they spend on the job.
- Data preparation
This involves cleaning and organizing the data you collect so it’s easier to use in training your AI model. It usually includes tasks like removing errors or resolving inconsistencies in your dataset.
Larger datasets naturally take longer to clean and prepare, so the cost will likely be higher. Also, error-prone data would require more cleaning efforts, increasing the potential costs.
Data preparation also involves transforming your data into a more suitable format for your AI model. For instance, converting text to numerical data. Additionally, if your project requires data from multiple sources, you must integrate data from various sources into a unified dataset.
You can choose from several data preparation tools. Each tool comes with different pricing models:
- Microsoft Power BI, for instance, starts at $20 monthly per user.
- Tableau prep is available at $15 monthly per user.
- IBM Cognos analytics at $10 monthly per user.
Of course, these tools have higher price plans, depending on your project needs.
The cost of acquiring, analyzing, and preparing data can run into millions of dollars for organizations. According to McKinsey, a mid-size company can spend over $250 million on data (this includes the cost of sourcing, preparation, architecture, and governance). See the data below:
Area | Description | Components | Typical Owner of Spend | Typical Spend, % of IT Spend | Example for a Midsize Financial Institution ($ million) |
Data sourcing | Cost associated with procuring data from customers; 3rd-party vendors, etc. | 3rd-party data | Head of business unit | 5–25% | 70–100 |
Data architecture | Cost associated with data infrastructure (procuring software, hardware) and data engineering (building and maintaining infrastructure) | Labor, infrastructure, and software | CIO | 8–15% | 90–120 |
Data governance | Cost of data-quality monitoring, remediation, and maintaining data-governance artifacts (e.g., data dictionary, data lineage) | Labor, software | Chief data officer | 2.5–7.5% | 20–50 |
Data consumption | Cost associated with data analysis and report generation (including spending on data access and cleanup) | Labor, software | Head of function or business unit | 5–10% | 60–90 |
Industries that don’t directly touch consumers (e.g., consumer packaged goods) spend a higher share (>20%) on data sourcing.
For midsize organizations with revenues of $5 billion to $10 billion and operating expenses of $4 billion to $6 billion. Absolute values vary by industry and size of the organization; e.g., absolute spend is, on average, higher for the telecommunications industry.
Source: McKinsey.
Using publicly available datasets can help reduce licensing fees. These datasets are available to analyze for free, although certain conditions may apply.
Additionally, being more specific about the type of data useful for your project can help you cut down costs from procuring irrelevant data. Also, acquire high-quality data that requires less cleaning to produce better results.
Labor and Expertise Costs
Businesses need skilled data science services, software developers, and machine learning engineers to create and implement AI successfully.
According to Indeed, the average salary for a data scientist is $124,109 per annum. For a software developer, it’s $120,068.
On average, a machine learning engineer would expect $162,699 yearly. So, building a small development team can cost more than $406,876 per year.
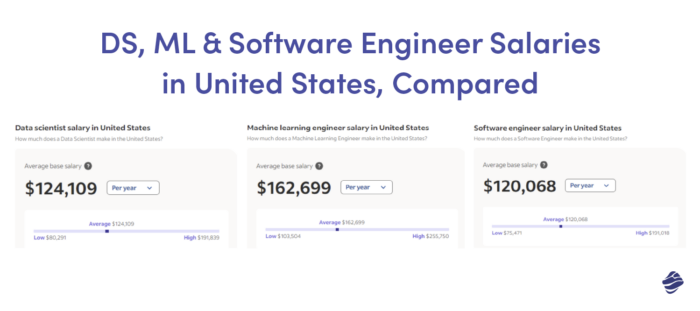
Hiring skilled professionals can become a significant expense in an AI project.
However, companies can consider hiring talent in lower-cost locations to manage labor costs effectively. They can also leverage open-source tools or pre-trained models( although these solutions may come with limitations).
Another incredible option is outsourcing to an AI app development company with the required talent, tools, and infrastructure.
How to Evaluate the ROI of AI Projects
Before investing in an AI project, assessing the immediate and long-term value your business will gain is imperative.
According to PWC, hard and soft ROIs are two benefits to expect from your AI investment.
Hard ROIs are quantitative benefits like time savings, productivity increases, cost savings, and revenue increases. Soft ROIs comprise qualitative benefits like better user experiences, skills retention, and agility. Keep these in mind when reviewing your ROIs.
You then need to consider hard and soft investments. Hard investments include the cash value of resources used in building the AI project. Soft investments include data, computing, storage, data training, and subject-matter experts.
See the illustration below:
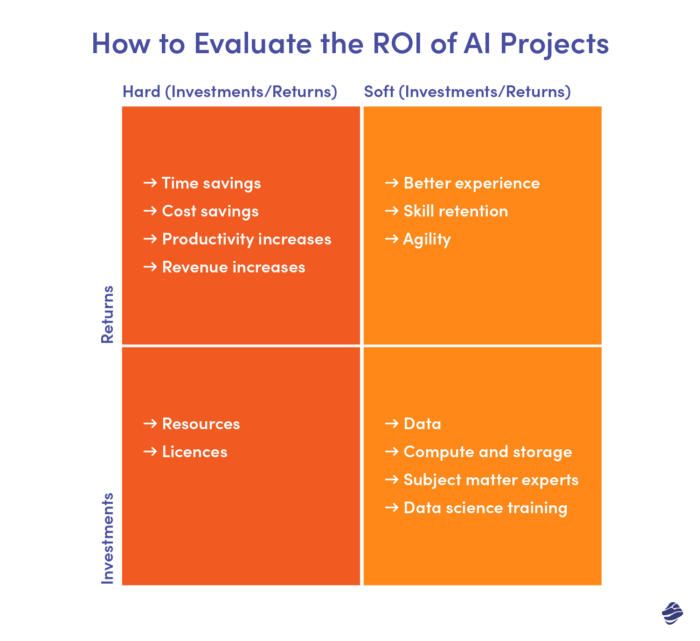
To evaluate your AI ROI, define success metrics that align with your overall business goals— paying attention to the qualitative metrics highlighted above. For instance, your success metrics may include:
- Improving customer satisfaction rating after implementing your AI software
- Enhanced employee satisfaction
- Reduced employee turnover
- Faster decision making
Ideally, your metrics will depend on the objective for starting the AI project. If you wanted to improve employee engagement, for instance, reduced employee turnover would be a great success metric to monitor.
Evaluate the total cost of ownership (TCO) of your AI project. This includes development, maintenance, infrastructure, and talent acquisition costs. Compare these costs to the projected benefits of the AI application.
Be sure to consider the uncertainty or hindrances that may come with gaining some qualitative benefits. For instance, the AI tool could encounter some errors or glitches, affecting its ability to perform specific tasks.
Monitor your success metrics throughout the project lifecycle to identify improvement areas and make necessary adjustments. This is also advisable because machine-learning models are likely to decline in performance over time. You may also need to feed more recent data into the system over time.
AI App Development Challenges for SMBs/SMEs
Studies reveal that SMEs may experience challenges like a lack of expertise or data availability when they start an AI project. This may lead to difficulty training AI models and limit the accuracy of their AI solutions.
Also, limited financial resources pose a barrier to successfully initiating or completing AI projects.
Additionally, AI implementation is often a continuous process of testing and modifying solutions. As a result, SMEs and SMBs may find it difficult to determine a clear ROI over time.
Since these organizations may be short on resources, it can also be tough to bear the cost of recovering from a failed AI adoption project.
Given these limitations, what feasible approach can SMEs use to implement successful AI projects?
- Instead of aiming for large, complex AI projects, SMEs/SMBs can target more specific and achievable AI solutions. This would allow for a more cost-effective venture.
- SMEs should also consider pre-trained AI models, tools, and services that run on a pay-as-you-go basis. This reduces upfront costs as there’s no need for extensive hardware infrastructure.
- They can also invest in training existing employees on relevant AI fundamentals. That’s one way to address internal knowledge gaps and facilitate AI adoption.
Finally, SMEs and SMBs can collaborate with technology providers to create AI solutions tailored to their specific needs and industry challenges. This should be a mutually beneficial partnership where the tech developer’s AI expertise is combined with the SME’s deep understanding of the business domain or target audience.
Case Studies of AI Development Projects
Before we round up our exploration of AI app development costs, let’s show you some successful AI development projects we’ve pulled off at Miquido:
- Nextbank
Nextbank provides a SaaS solution offering a white-label mobile banking app that partner banks can customize with their branding. The focus of the app development project was building a cost-effective, secure, and user-friendly platform.
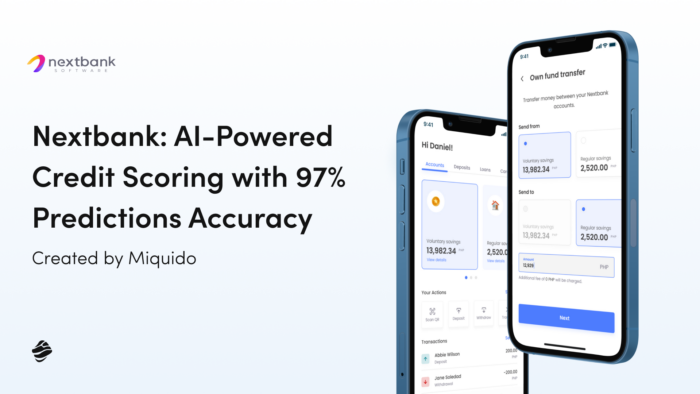
One notable feature of the Nextbank App is AI-powered credit scoring. This feature uses machine learning algorithms to prepare a list of potential borrowers likely to repay loans. Other key functionalities of the Nextbank app include seamless user onboarding, biometric authentication, and QR code payments.
Some key challenges involved with building the app included ensuring seamless interactions, maintaining strong security, and designing for customization. Partnering with Miquido helped Nextbank optimize costs on various levels, including developing and maintaining the application.
- Social Bee
Social Bee is an AI-powered tool that translates complex legal texts within seconds. For this project, the challenge involved building a cost-effective app that maintains cultural relevance in AI translations, accommodates language diversity, and performs optimally.
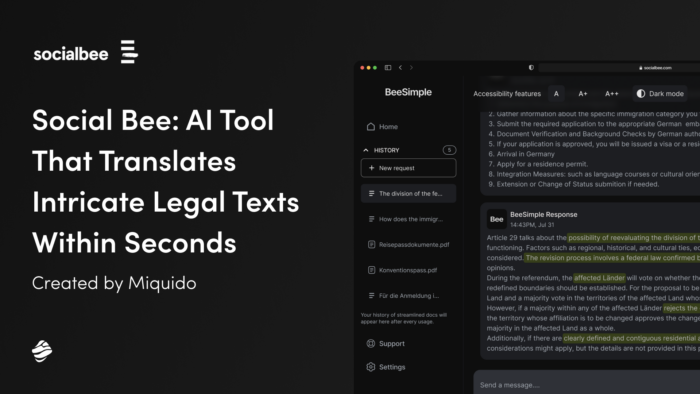
Miquido created a scalable proof of concept using the OpenAI API, integrating it with GPT 3.5. We also developed a proof of concept designed to accommodate future iterations and modifications.
Other major AI projects we’ve been involved in include:
- Nolej – an AI-powered platform that allows teachers and instructional designers to quickly generate interactive eLearning materials.
- Caliber – a human resource AI tool that helps streamline candidate scoring.
- Youmap AI – for AI-enhanced user content generation.
- Verseo – an internal knowledge base powered by AI.
We focused on tailoring solutions to unique project requirements and streamlining the development process. This resulted in cost-effective solutions that allowed our clients to maximize available resources.
Selecting the right AI partners and tools is crucial for the success of your AI project. However, with the myriad of vendors littered across the Gen AI marketplace, it may be tough to decide on the right choice for you.
Generally, the AI Vendor landscape is categorized into four major groups:
- Foundational Large Language Model Providers
They offer access to pre-trained foundation models or LLMs, which are trained on massive datasets and can perform various tasks, such as text generation, translations, and code completion. Examples include Open AI, Microsoft Azure AI, and Google AI.
The cost of accessing pre-trained LLMs is usually high as they involve licensing fees or subscription costs based on usage. Fine-tuning these models may also attract additional costs. However, using pre-trained LLMs helps reduce development time and minimize in-house recruitment needs.
- Infrastructure providers
They offer the hardware and software infrastructure needed to train and run generative AI models. That includes cloud computing, specialized hardware, and data storage solutions.
Infrastructural services typically run on a pay-as-you-go price model. That allows you to save on upfront investments and scale your infrastructure based on operational needs.
Examples include Amazon Web Services (AWS), Microsoft Azure, and Google Cloud Platform.
- Software providers
They offer user-friendly tools and frameworks specially tailored for generative AI development. This helps to streamline data preparation, model training, and deployment.
Pricing is subscription-based, and the costs often depend on the chosen software or usage level. Examples are NVIDIA DGX, Paperspace Gradient, and Gradio. These solutions can reduce the need for custom coding and save development costs in the long run.
- IT service providers
They mainly offer consulting, implementation, and support for generative AI projects. These service providers include companies like IBM, Deloitte, and Accenture. Costs are usually based on the scope of services provided.
Here’s a simple pictorial breakdown of the AI vendor landscape:
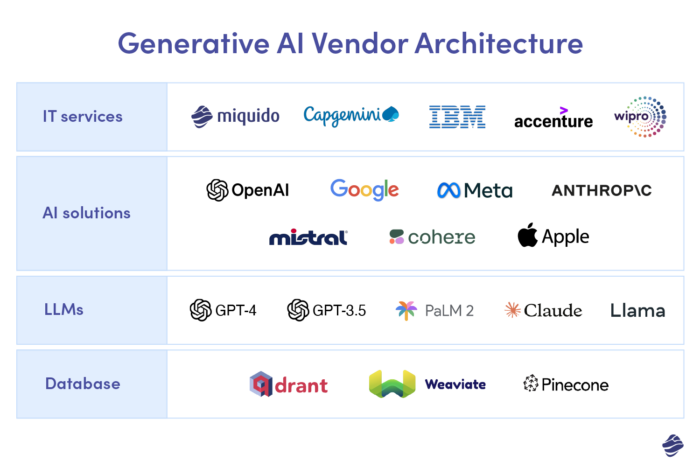
When choosing vendors, consider factors like expertise, experience, and flexibility. Also, ensure the vendor has a portfolio that matches your project requirements and desired functionalities.
Additionally, the vendor should have robust data security practices, which is one of the significant AI trends in mobile apps and solutions. Choose a vendor that adheres to relevant privacy regulations and prioritizes transparent communication.
Finally, ensure client support is available throughout the project’s lifecycle.
Future Cost Trends in AI Development
AI development has come a long way. Early AI developments focused on solving problems using logic and rule-based systems. Then, machine learning solutions, which use algorithms that learn from data, came into the picture. This was followed by deep learning techniques that used complex artificial neural networks to improve natural language processing.
Presently, AI powers real-world applications for a wide range of purposes, and further advancements are underway. For instance, we have state-of-the-art models like GPT-4, which can conveniently process texts, images, and videos.
So, what does the future look like for AI development?
- Transition to smaller language models
According to the MIT Technology Review, recent efforts by AI providers like Google and Open AI aim to develop simpler platforms that allow the customization of powerful language models. These modifications can help people create their chatbots that cater to specific needs.
That’s only one scenario in the transition from LLMs to smaller language models that are less resource-intensive and yield better results.
- Affordable and accessible AI frameworks
Open-source libraries will likely become more efficient in training and running AI models. This will lead to the development of AI frameworks that are more affordable and accessible to a wider range of users, including SMEs and SMBs.
- Specialized AI development platforms
Specialized AI development platforms are likely to come into the picture. These platforms offer tailored solutions for select industries or use cases, potentially minimizing operational costs for businesses in those niches.
Overall, the future trends in AI development point to more cost-effective solutions for businesses.
In Closing: How Much Does it Cost to Develop an AI App?
AI solutions are applicable in a wide range of business functions. Examples include basic solutions like automation of repetitive tasks, personalized product recommendations, inventory management, and accurate financial predictions.
However, you must consider the costs and benefits before taking on major AI app development projects.
Some specific costs to keep in mind include data acquisition and preparation, labor, expertise, and infrastructure. These costs will vary depending on the complexity of your specific AI project.
Apart from the costs, it’s also important to understand the various vendors in the AI market and which services are relevant to your direct project needs. Qualitative and quantitative ROIs are also vital items to assess.
With that in mind, you should be able to plan for a more budget-friendly AI project without compromising on quality.