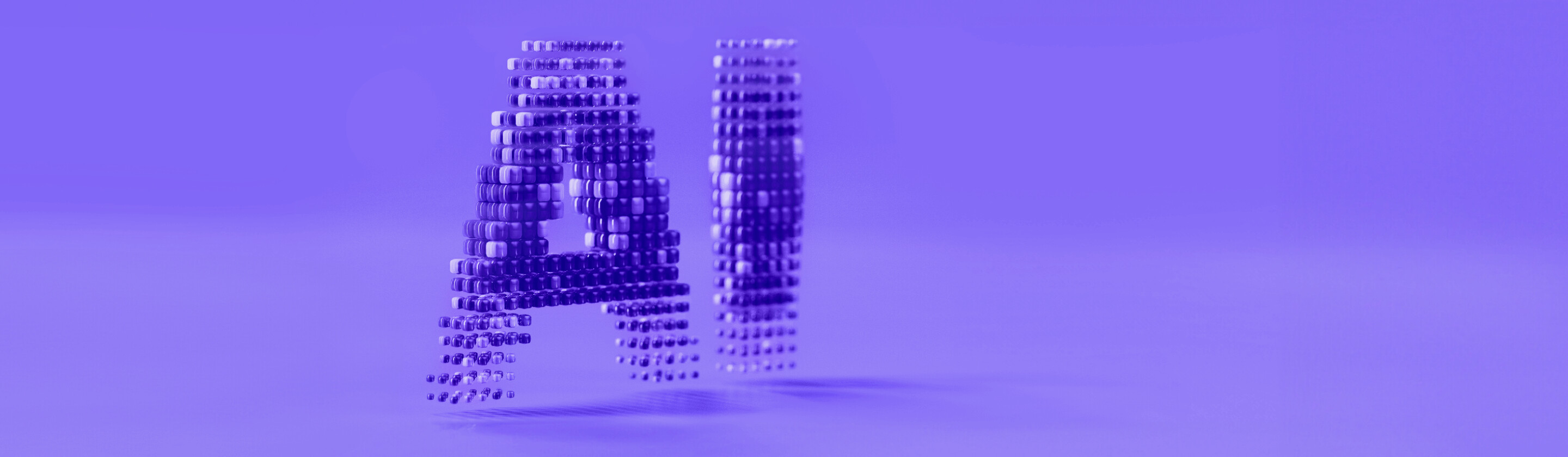
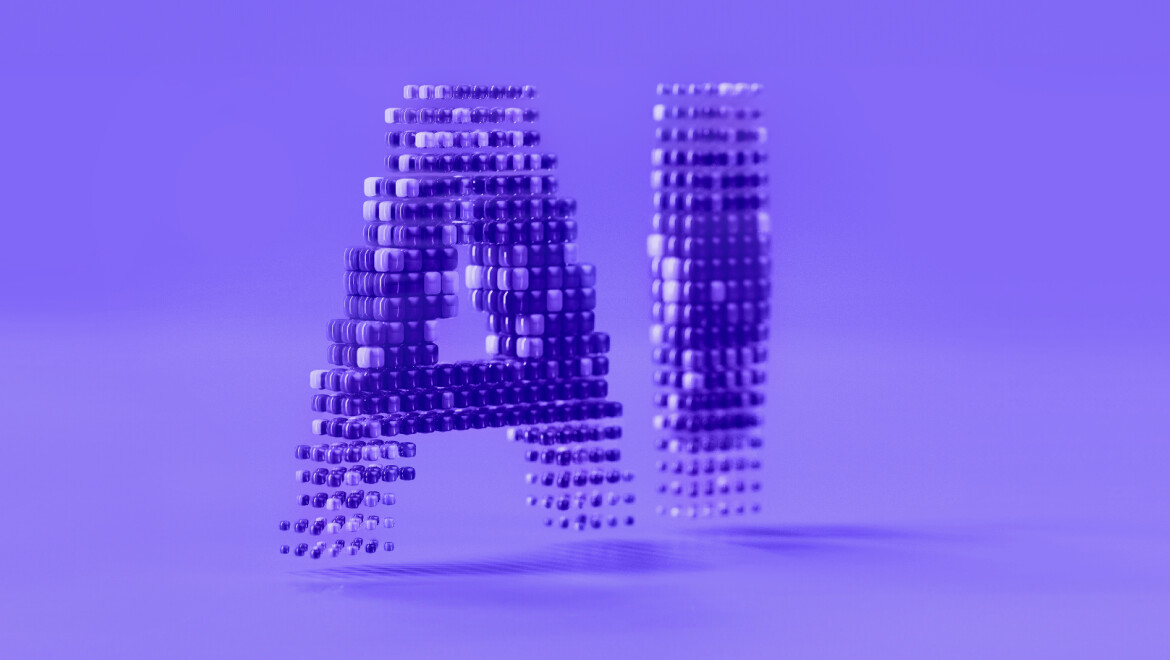
What is AI Bias?
What is AI Bias?
AI bias refers to the systematic unfairness or discrimination present in artificial intelligence systems. It happens when an AI system produces prejudiced or unfair results, typically because of the data it was trained on or the way it was designed. These biases can manifest in many ways, affecting decisions in areas like hiring, credit approvals, law enforcement, and healthcare. The critical issue lies in how AI models learn from historical data, which can reflect societal inequalities. If the data contains skewed patterns, the AI will inherit those biases, leading to unequal treatment across different demographics. This raises serious ethical concerns, as AI systems often impact decisions with real-world consequences.
Why Does AI Bias Happen?
To understand what is AI bias, it’s important to recognize that bias can emerge from several factors:
- Biased Data: Most AI systems learn from large datasets that can contain implicit human biases. If the data includes historical inequalities, like underrepresentation of certain groups, the AI will adopt those patterns, making unfair decisions.
- Flawed Algorithms: Algorithms themselves can be designed in ways that inadvertently introduce bias. This happens if the metrics they use prioritize certain groups over others. For example, an AI hiring tool might give more weight to certain qualifications that are typically associated with a particular demographic, leading to biased outcomes.
- Lack of Diverse Input: Bias can also be introduced if the AI development team lacks diverse perspectives. If only a narrow group of people design and train the system, their own unconscious biases might be built into the model.
- Feedback Loops: Once an AI system starts making biased decisions, it can create a feedback loop where these decisions reinforce the existing bias. For instance, if a predictive policing algorithm focuses on certain neighborhoods, it may lead to increased surveillance and arrests in those areas, further justifying the algorithm’s initial bias.
AI Bias Example
A prominent AI bias example occurred in recruitment algorithms. A major tech company developed an AI to sift through resumes for top candidates. However, the system was trained on data from a predominantly male workforce. As a result, the AI started downgrading resumes from women, especially those that mentioned women’s colleges or activities like women’s organizations. This example of AI bias demonstrates how skewed data can result in discriminatory outcomes.
More Examples of Bias in AI
- Facial Recognition: One of the most concerning examples of AI bias involves facial recognition systems. Studies have shown that these systems are less accurate in identifying people with darker skin tones, particularly Black women. A study conducted by MIT found that commercial facial recognition technologies misidentified Black women at rates of up to 35%, while the error rate for white males was less than 1%. This stark discrepancy is a clear example of bias in AI, raising concerns about the use of facial recognition technology in areas like law enforcement.
- Healthcare: In healthcare development, AI algorithms have been found to be biased against minority groups. For example, an algorithm used to prioritize patients for care was found to systematically underestimate the medical needs of Black patients. The system relied on healthcare spending data, and since Black patients historically spent less on healthcare (due to unequal access), the algorithm allocated fewer resources to them. This is a serious example of AI bias that can lead to life-threatening consequences.
- Predictive Policing: Another example of bias in AI can be found in predictive policing tools. These systems use historical crime data to predict future criminal activity. However, because certain communities are historically over-policed, the algorithm often directs more police resources to those areas, reinforcing existing biases. This not only increases the likelihood of arrests in certain demographics but also perpetuates the cycle of bias in law enforcement.
- Credit Scoring and Loan Approvals: AI systems used for credit scoring or loan approvals can also reflect bias. An AI bias example in the financial sector showed that minority applicants were disproportionately denied loans. The AI was trained on historical lending data, which included biases against certain racial or socioeconomic groups. As a result, these groups faced higher rejection rates, even when they had similar financial profiles to other applicants.
Addressing AI Bias
To fully grasp what is AI bias and its implications, it’s crucial to develop strategies to mitigate it:
- Data Diversity: One of the most important ways to reduce bias is by using diverse datasets. When training AI, the data should be representative of the entire population to avoid favoring any particular group.
- Bias Auditing: Regular audits of AI systems can help detect and mitigate biases. Independent reviews can be conducted to ensure transparency and fairness in how the AI is making its decisions.
- Transparent Algorithms: Understanding what is AI bias also involves promoting transparency in how AI systems work. By making algorithms more transparent, developers and users can understand the reasoning behind the AI’s decisions, allowing for accountability and corrections where necessary.
- Human Oversight: Keeping humans in the loop is essential, especially in sensitive areas like healthcare and criminal justice. Human oversight ensures that AI systems don’t operate in a vacuum and that potentially biased outputs can be corrected before they cause harm.
In conclusion, what is AI bias? It’s a fundamental issue that impacts the fairness and accuracy of AI systems. As AI becomes more integrated into everyday decision-making, addressing and preventing bias is critical to ensuring that these technologies work equitably for everyone. Understanding examples of AI bias in areas like hiring, law enforcement, healthcare, and finance helps highlight the importance of creating unbiased AI systems.
Ready to discover more terms?