- Home
- AI Glossary
- Foundation Models in Generative AI
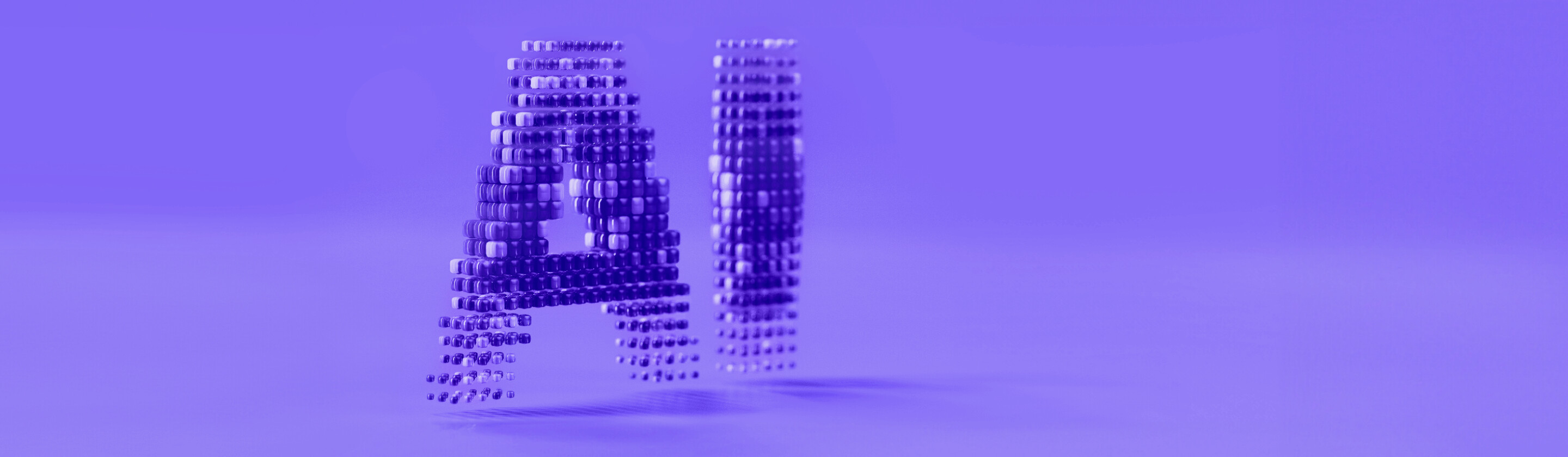
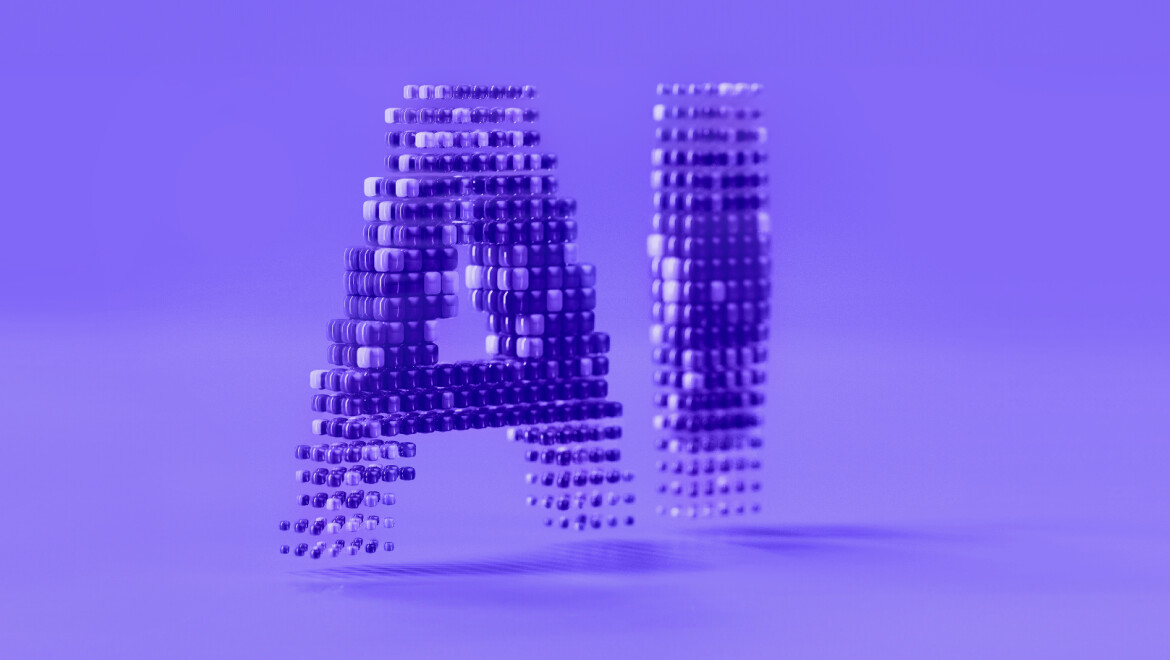
Foundation Models in Generative AI
Foundation Models in Generative AI
What are foundation models in Generative AI?
Foundation models in generative AI are large, pre-trained models that serve as a base for a wide variety of AI applications. These foundation models differ from other AI models, such as narrow AI models, by providing a versatile basis that can be adapted for various specialized tasks. They are built on massive datasets, often using billions of data points, and are trained through extensive computing power to learn a wide array of patterns, representations, and relationships in language, images, or other data forms.
Foundation models can then be fine-tuned or adapted to perform specific tasks, making them versatile tools in generative AI. Foundation models work by leveraging underlying technologies like transfer learning and scale, which enable their adaptability to multiple data types and ensure their essential role in the future of generative AI in enterprises.
What is a Foundation Model in Generative AI?
In simple terms, a foundation AI model is a large-scale machine learning model that acts as a foundation for more specialized AI systems. These models are pre-trained on diverse datasets, which enables them to understand complex data patterns and relationships. After this general training phase, foundation models can be fine-tuned with smaller, domain-specific datasets to perform specific tasks—such as text generation, translation, or image creation. Notably, models like GPT (for text) and DALL-E (for images) are popular examples of foundation models in generative AI.
Key Characteristics of Foundation Models
- Scale and Versatility: Foundation models are built on a massive scale, typically consisting of billions of parameters. This extensive training enables them to adapt to a wide range of downstream tasks, from language processing to visual recognition. Deep learning models contribute significantly to the development of foundation models, particularly in natural language processing, computer vision, and audio processing.
- Pre-Training on Diverse Data: By training on large and varied datasets, these models develop a generalized understanding of data structures, which allows them to perform multiple tasks without needing task-specific training data from scratch.
- Transferability: Foundation models are designed to be adapted for specific applications, allowing developers to fine-tune them on smaller datasets for particular tasks without retraining from the ground up.
Examples of Foundation Models
GPT Models (by OpenAI): GPT-3 and GPT-4 are large language models trained on diverse text data. They can generate human-like responses, create essays, summarize text, and perform various other NLP tasks.
BERT (by Google): BERT, another language model, excels in understanding context and can be fine-tuned for tasks like sentiment analysis or question-answering.
DALL-E and Stable Diffusion: These image generation models can create realistic images from textual descriptions, trained on massive datasets of image-text pairs to learn associations between words and visual elements.
Applications of Foundation Models in Natural Language Processing and Generative AI
Foundation models enable a wide range of applications across industries:
- Natural Language Processing (NLP): Foundation models are widely used for text generation, summarization, translation, and chatbots, as they provide a base for developing conversational AI and automated writing tools. Platforms and tools facilitate the deployment of machine learning models for NLP tasks, making it easier to build, deploy, and share these models effectively.
- Image and Video Generation: Models like DALL-E can create images from text prompts, serving as tools in design, marketing, and content creation.
- Medical Diagnostics: Foundation models adapted for medical imaging can assist in diagnosis by analyzing complex images and recognizing patterns indicative of certain health conditions.
- Scientific Research: Foundation models aid in data analysis, molecule design, and research publication summarization, accelerating scientific discovery across various domains.
Advantages and Limitations of Foundation Models
Advantages:
- Efficiency: By pre-training on extensive data, foundation models reduce the need for task-specific data and training, allowing faster development.
- Adaptability: These models can be customized for numerous applications, making them highly flexible.
- State-of-the-Art Performance: Due to their scale and depth, foundation models often achieve high accuracy and effectiveness in diverse applications.
Limitations:
- High Resource Demand: Training foundation models requires significant computational resources, which can limit access.
- Risk of Bias: Since these models are trained on vast datasets, they may inadvertently learn and propagate biases present in the data.
Why Foundation Models are Important in Generative AI
Foundation models represent a transformative approach in generative AI, enabling rapid, flexible adaptation to new tasks and reducing the time and cost required for developing AI applications. By providing a common starting point, they accelerate AI innovation, making it easier for researchers and companies to create sophisticated tools tailored to specific needs. As AI research continues to advance, foundation models will likely drive the development of even more intelligent, versatile, and accessible AI applications across industries.
Ready to discover more terms?