- Home
- AI Glossary
- Data Sampling Definition
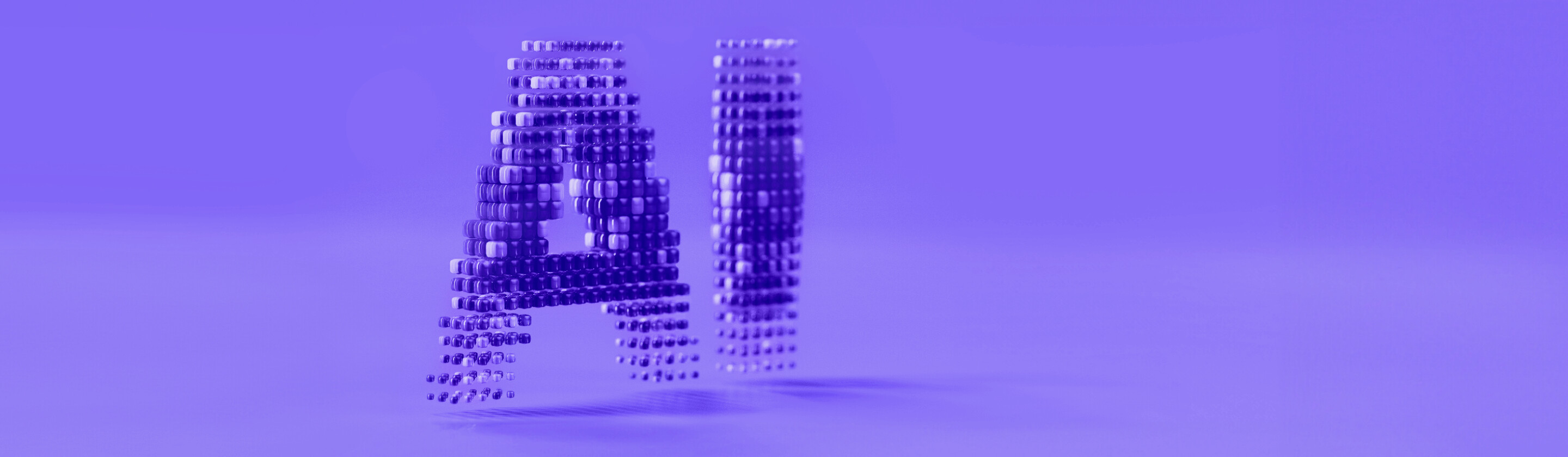
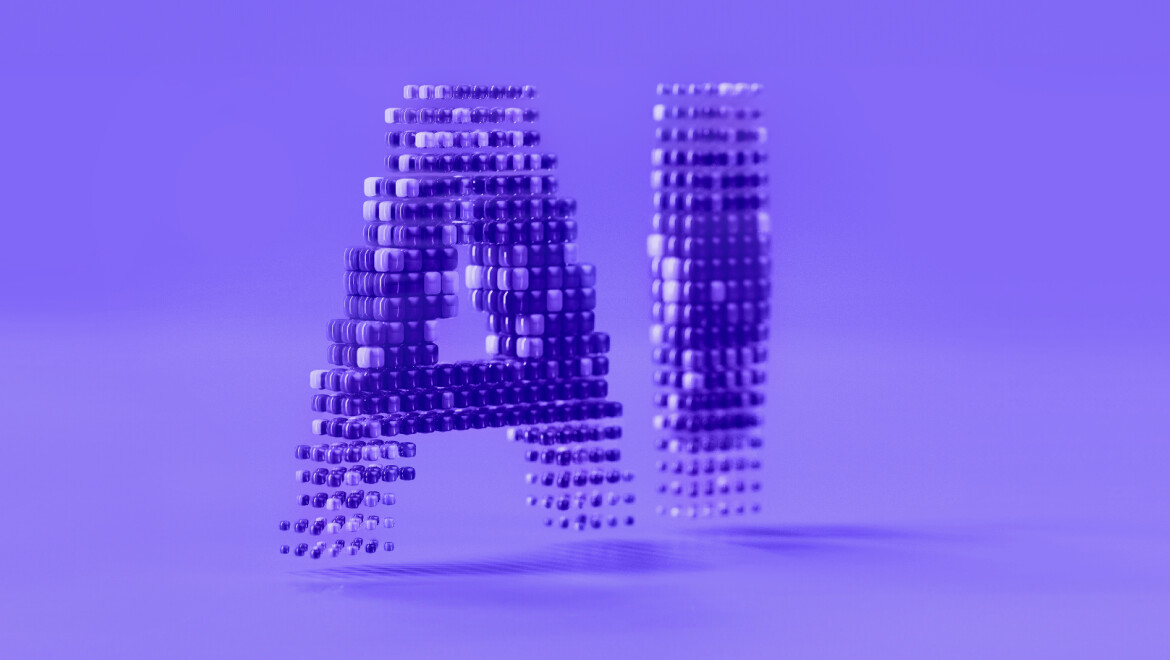
Data Sampling Definition
Data Sampling Definition
What is Data Sampling?
Data sampling is a statistical technique that involves selecting, analysing, and drawing conclusions from a smaller subset of data from a larger data set called a population. Data sampling is a method that allows researchers to make inferences about a population without having to study the whole population. This can be time-consuming, costly, or even impossible.
Data sampling is used in various contexts, from scientific research to business analytics. Data samples serve as practical representations of a larger group. Data sampling methods can be either probabilistic or non-probabilistic. Probabilistic sampling ensures that each member of the population has a known chance of being included, while non-probabilistic sampling does not involve random selection.
Data Sampling Meaning Explained
Data sampling is essential because it offers a manageable and cost-effective way to obtain insights about a population. Pollsters use data sampling to predict election results by surveying a small group of people that reflect the larger voting populace. Similarly, quality control in manufacturing often involves data sampling to assess whether products meet certain standards without inspecting every single item.
Understanding data sampling is essential for anyone working with data science development. It ensures that the conclusions drawn are based on representative and unbiased information, thus increasing the reliability of the results. Data sampling remains vital for extrapolating meaningful insights from smaller, manageable datasets to inform more significant trends and patterns.
Transformative Advances in Data Sampling
Data sampling is experiencing transformative advances, particularly in survey research methodologies. A special issue from the Journal of Survey Statistics and Methodology highlights this transformation and the ongoing debate over the merits and costs of probability versus non-probability sampling methods. It introduces empirical evidence and conceptual approaches to sampling strategies and investigates their applications in various fields, like healthcare app development and big data. This work also explores innovative techniques like using non-probability samples as informative priors in Bayesian models and applying quasi-random approaches to naturalistic driving data. These advancements underscore the evolving nature of data sampling methods in addressing the complexities of today’s data-rich world.
Ready to discover more terms?