- Home
- AI Glossary
- AI Token Definition
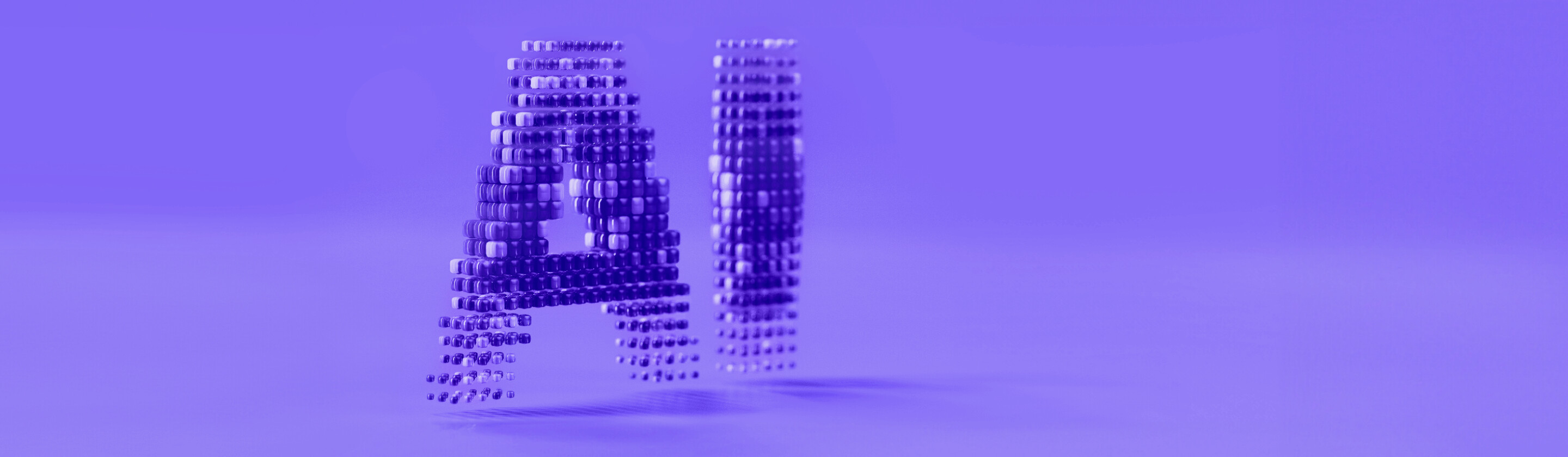
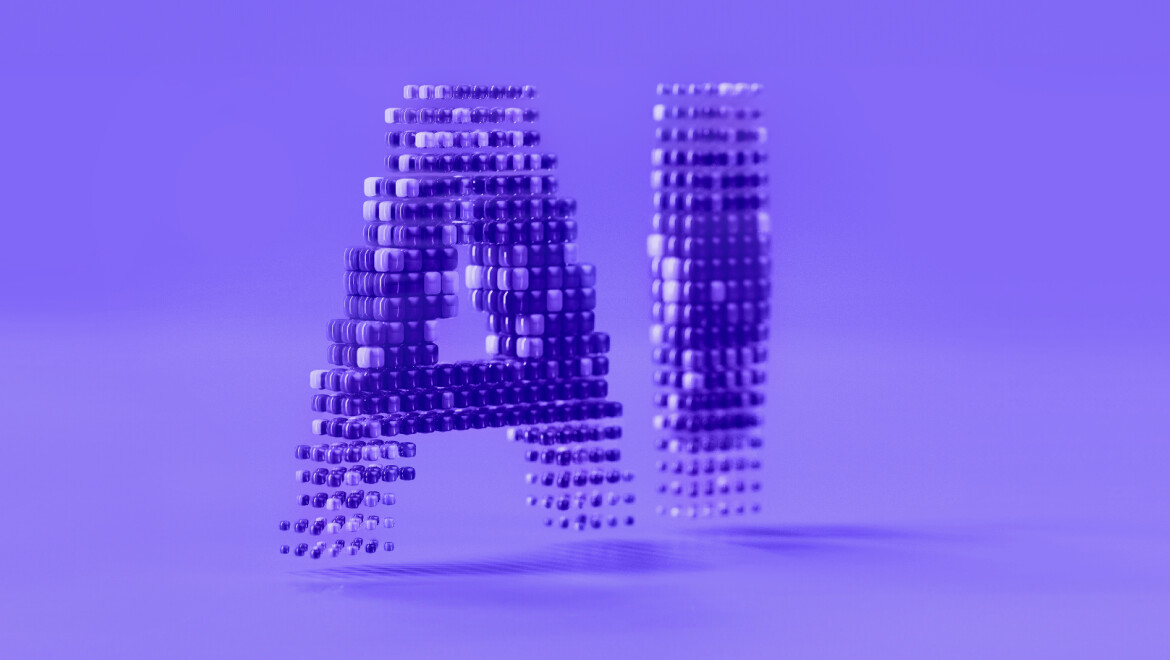
AI Token Definition
AI Token Definition
What is a Token in AI?
In the field of AI, a token is a fundamental unit of data that is processed by algorithms, especially in natural language processing (NLP) and machine learning services. A token is essentially a component of a larger data set, which may represent words, characters, or phrases. For example, when processing text, a sentence is divided into tokens, where each word or punctuation mark is considered a separate token. This process of tokenisation is a crucial step in preparing data for further processing in AI models.
Tokens are not restricted to text alone. They can represent various data forms and play a crucial role in AI’s ability to understand and learn from them. For instance, in computer vision, a token may denote an image segment, like a group of pixels or a single pixel. Similarly, in audio processing, a token might be a snippet of sound. This flexibility of tokens makes them essential in AI’s ability to interpret and learn from different data forms.
The Importance of Token in AI
Tokens play an essential role in AI, particularly in machine learning models that involve language tasks. In such models, tokens serve as inputs for algorithms to analyse and learn patterns. For instance, in a chatbot development, each word in the user’s input is treated as a token, which helps the AI understand and respond appropriately.
In advanced AI models like transformers, tokens are even more crucial. These models process tokens collectively, enabling the AI to understand context and nuances in language. This understanding is critical for tasks like translation, sentiment analysis, and content generation.
In summary, tokens are basic yet powerful units of data in AI development. They are foundational elements that allow algorithms to process and learn from various data types, such as text, images, and sounds. The token AI concept is crucial for various AI applications, from simple text processing to complex tasks involving understanding context and subtleties in human language.
Ready to discover more terms?