- Home
- AI Glossary
- AI Hallucination Definition
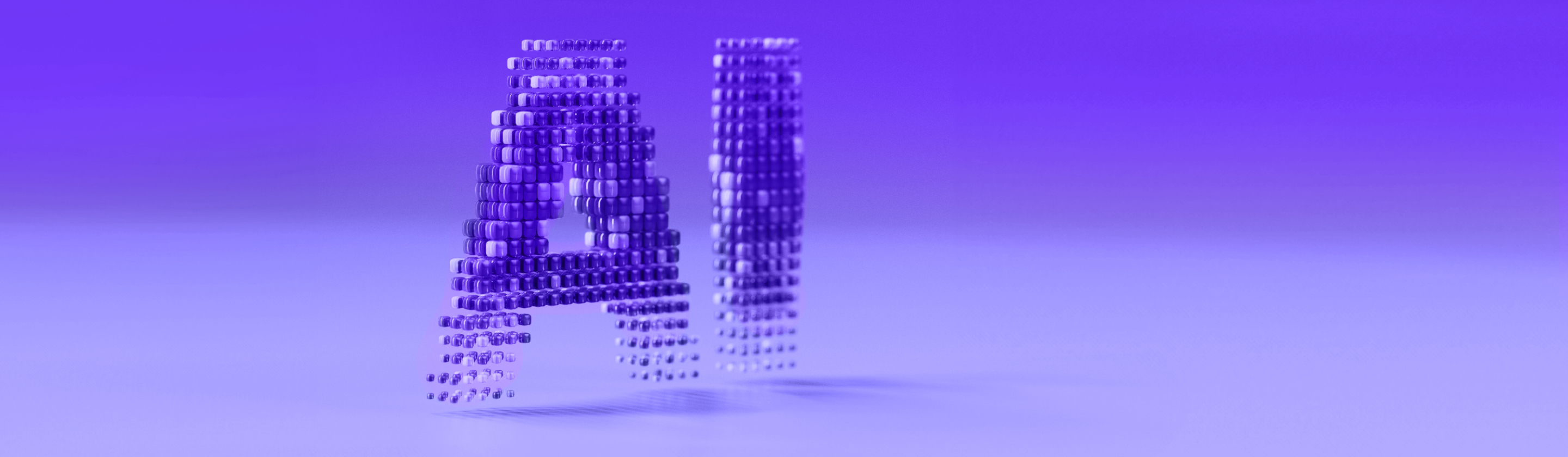
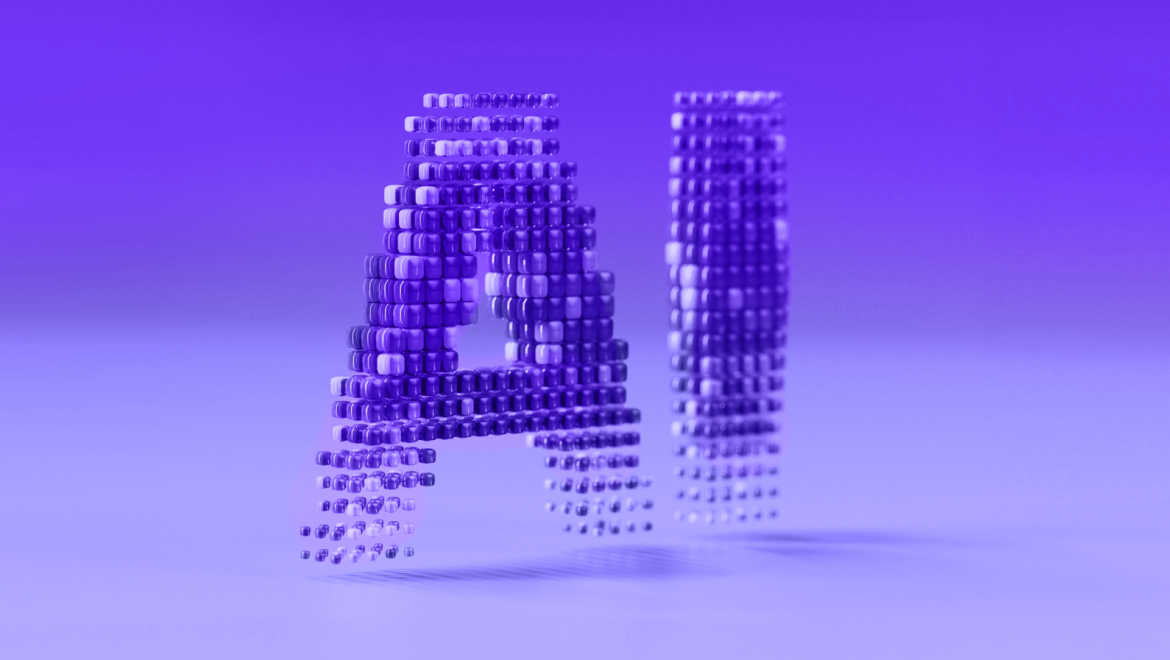
AI Hallucination Definition
AI Hallucination Definition
AI Hallucination Definition
What Is an AI Hallucination?
AI hallucination refers to a scenario where generative AI tools produce erroneous or misleading outputs that appear to be based on factual data but are fabricated. The degree of hallucination can vary from minor inaccuracies to the creation of entirely fictitious data that appears authentic. The underlying reasons behind AI hallucinations are complex and can be attributed to various factors, including:
- Training Data Sources: Generative AI models are trained on vast amounts of internet data, including accurate and inaccurate content and societal and cultural biases. These models simply reproduce patterns in their training data without discerning the truth, leading to the replication of any falsehoods or biases present in the data.
- Limitations of Generative Models: Generative AI models aim to produce plausible content rather than verify truth. Any accuracy in their outputs is often coincidental. This can result in content that sounds plausible but is inaccurate.
- Inherent Challenges in AI Design: Even if trained on accurate data, generative AI models could still produce inaccurate content because they combine patterns in unexpected ways. These models do not inherently differentiate between what’s true and what’s not.
How To Deal With Hallucinations In Generative AI
Preventing AI hallucinations requires continuous research and human supervision, including improving the training process, enhancing training data quality, creating efficient algorithms, and following the “human-in-the-loop” approach.
AI and Hallucinations Controversies
The term “AI hallucination” has caused disagreement among experts. Some, like Usama Fayyad from Northeastern University, disagree with the term. They suggest that it inaccurately represents AI errors by ascribing intent or consciousness to the AI models. These models function as advanced autocomplete tools that generate plausible content based on patterns observed in their training data, not to verify the truth. Thus, their outputs might sound convincing but can be entirely inaccurate. This issue is further complicated by the fact that the output heavily depends on the prompt given to the AI. Even slight adjustments can result in vastly different outcomes.
Ready to discover more terms?